Dissociating curiosity-driven exploration algorithms.
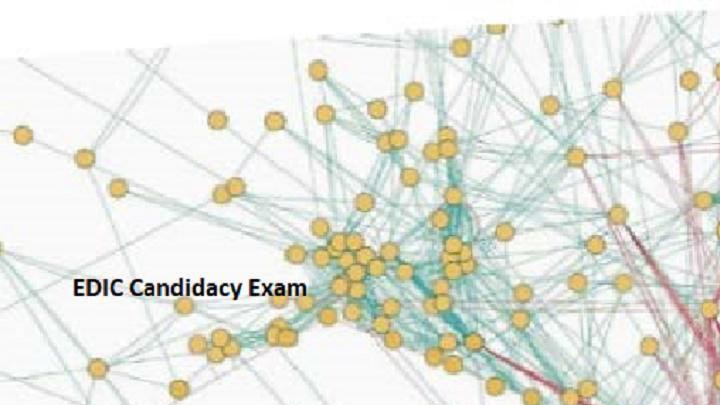
Event details
Date | 02.05.2024 |
Hour | 09:00 › 11:00 |
Speaker | Lucas Gruaz |
Location | |
Category | Conferences - Seminars |
EDIC candidacy exam
Exam president: Prof. Martin Jaggi
Thesis advisor: Prof. Wulfram Gerstner
Co-examiner: Prof. Nicolas Flammarion
Abstract
Exploration is a fundamental concept both in Reinforcement
Learning (RL) and human behavior. In RL, exploration
involves the agent actively seeking information about
its environment to discover optimal strategies for maximizing
rewards. Similarly, in human behavior, exploration manifests
as curiosity, experimentation, and risk-taking, all of which
contribute to learning and adaptation. By exploring the unknown,
both RL agents and humans can discover novel solutions, adapt
to changing circumstances, and ultimately improve their performance
and understanding of the world around them. Various
methods have been developed to encourage exploration in RL
agents. Their specificity and application scenarios are varied, and
their similarity with exploration strategies observed in humans
remains unclear. In this proposal, we review three paper related
to this question. The first paper gives an overview of exploration
techniques in deep RL, the second paper shows an example of
a successful application of such techniques, and the third paper
explore human exploratory behavior, serving as a starting point
to assess differences with previously introduced techniques.
Background papers
Exam president: Prof. Martin Jaggi
Thesis advisor: Prof. Wulfram Gerstner
Co-examiner: Prof. Nicolas Flammarion
Abstract
Exploration is a fundamental concept both in Reinforcement
Learning (RL) and human behavior. In RL, exploration
involves the agent actively seeking information about
its environment to discover optimal strategies for maximizing
rewards. Similarly, in human behavior, exploration manifests
as curiosity, experimentation, and risk-taking, all of which
contribute to learning and adaptation. By exploring the unknown,
both RL agents and humans can discover novel solutions, adapt
to changing circumstances, and ultimately improve their performance
and understanding of the world around them. Various
methods have been developed to encourage exploration in RL
agents. Their specificity and application scenarios are varied, and
their similarity with exploration strategies observed in humans
remains unclear. In this proposal, we review three paper related
to this question. The first paper gives an overview of exploration
techniques in deep RL, the second paper shows an example of
a successful application of such techniques, and the third paper
explore human exploratory behavior, serving as a starting point
to assess differences with previously introduced techniques.
Background papers
- Paper 1: Ladosz et al. “Exploration in deep reinforcement learning: A survey”. Information Fusion, 85:1–22, Sept. 2022. https://www.sciencedirect.com/science/article/pii/S1566253522000288
- Paper 2: Badia et al. “Agent57: Outperforming the Atari Human Benchmark”. In Proceedings of the 37th International Conference on Machine Learning, pages 507–517. PMLR, Nov. 2020. https://arxiv.org/abs/2003.13350
- Paper 3: Brändle et al. “Empowerment contributes to exploration behaviour in a creative video game”. Nature Human Behaviour, 7(9):1481–1489, Sept. 2023. https://www.nature.com/articles/s41562-023-01661-2
Practical information
- General public
- Free
Contact
- edic@epfl.ch