Model predictive control of stochastic linear discrete-time systems
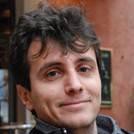
Event details
Date | 29.05.2015 |
Hour | 10:15 |
Speaker | Marcelo Farina |
Location | |
Category | Conferences - Seminars |
The problem of designing robust deterministic model predictive control (MPC) schemes has nowadays many solutions. However, the available approaches can
be computationally demanding, since they either require the solution to difficult on-line min-max optimization problems, or the off-line computation of polytopic robust positive invariant sets. In addition they are conservative, mainly because they (implicitly or explicitly) rely on worst-case approaches. If the uncertainties or the state and control disturbances are characterized as stochastic processes, constraints should be reformulated in a probabilistic framework, and the available knowledge on the characteristics of the process noise (e.g., probability density function) should be properly accounted for in the design phase.
These reasons have motivated the development of MPC algorithms for systems affected by stochastic noise and subject to probabilistic state and/or input constraints.
In this seminar we discuss and classify some possible approaches addressing this issue in the literature. Then, we describe a recently-proposed MPC algorithm for linear discrete-time systems affected by a possibly unbounded additive noise and subject to probabilistic constraints and its properties.
Application examples are finally discussed.
Bio: Marcello Farina received the Laurea degree in Electronic Engineering in 2003 and the PhD degree in Information Engineering in 2007, both from the Politecnico di Milano. In 2005 he was visiting student at the Institute for Systems Theory and Automatic Control, Stuttgart, Germany. He is presently Associate Professor at Dipartimento di Elettronica, Informazione e Bioingegneria, Politecnico di Milano. His research interests include distributed and decentralized state estimation and control, stochastic model predictive control, and applications, e.g., mobile robots, sensor networks, and energy supply systems.
be computationally demanding, since they either require the solution to difficult on-line min-max optimization problems, or the off-line computation of polytopic robust positive invariant sets. In addition they are conservative, mainly because they (implicitly or explicitly) rely on worst-case approaches. If the uncertainties or the state and control disturbances are characterized as stochastic processes, constraints should be reformulated in a probabilistic framework, and the available knowledge on the characteristics of the process noise (e.g., probability density function) should be properly accounted for in the design phase.
These reasons have motivated the development of MPC algorithms for systems affected by stochastic noise and subject to probabilistic state and/or input constraints.
In this seminar we discuss and classify some possible approaches addressing this issue in the literature. Then, we describe a recently-proposed MPC algorithm for linear discrete-time systems affected by a possibly unbounded additive noise and subject to probabilistic constraints and its properties.
Application examples are finally discussed.
Bio: Marcello Farina received the Laurea degree in Electronic Engineering in 2003 and the PhD degree in Information Engineering in 2007, both from the Politecnico di Milano. In 2005 he was visiting student at the Institute for Systems Theory and Automatic Control, Stuttgart, Germany. He is presently Associate Professor at Dipartimento di Elettronica, Informazione e Bioingegneria, Politecnico di Milano. His research interests include distributed and decentralized state estimation and control, stochastic model predictive control, and applications, e.g., mobile robots, sensor networks, and energy supply systems.
Practical information
- Expert
- Free