Multi-Person Monocular 3D Pose Estimation Using Deep Neural Networks
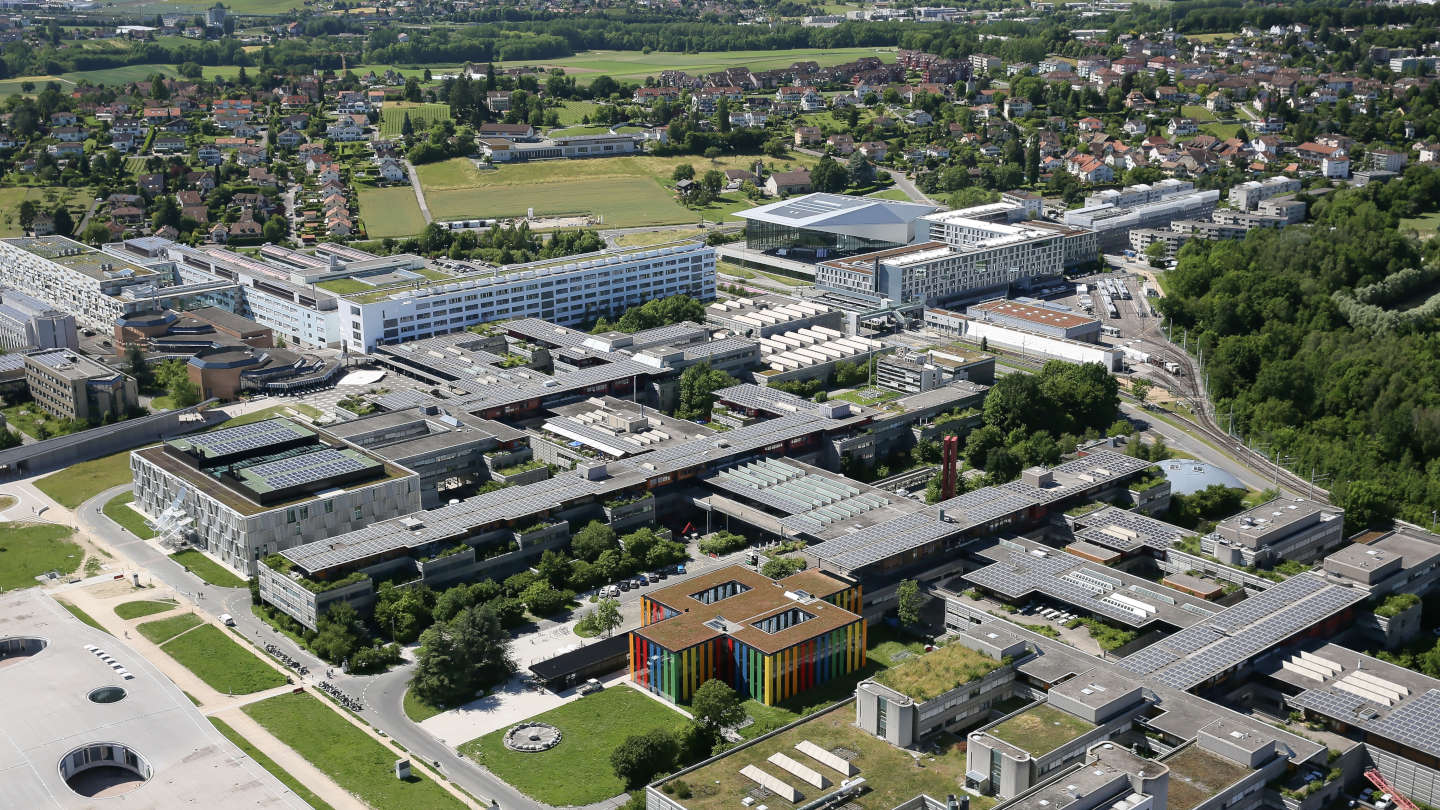
Event details
Date | 27.06.2017 |
Hour | 10:30 › 12:30 |
Speaker | Isinsu Katircioglu |
Location | |
Category | Conferences - Seminars |
EDIC candidacy exam
Exam president: Prof. Mark Pauly
Thesis advisor: Prof. Pascal Fua
Thesis co-advisor: Dr. Mathieu Salzmann
Co-examiner: Prof. Sabine Süsstrunk
Abstract
Recovering 3D pose of multiple interacting subjects in unconstrained environments is highly challenging due to strong partial occlusions and ambiguities caused by the close proximity of individuals. In this work, we address the problem of multi- person 3D pose estimation from monocular RGB images using deep neural networks. First we discuss three related methods that tackle different aspects of our problem: a framework for 3D human pose prediction using shape context matching, a deep learning model for multi-person 2D pose estimation that is cast as an integer linear programming problem and an approach for identifying collective activities from videos containing a group of interacting people. Finally, we introduce our deep learning method that regresses individual 3D poses from heatmaps of 2D joint locations and feeds them to a dependency network to model the correlations between them. We evaluate the performance of our approach on a new boxing dataset.
Background papers
DeeperCut: A Deeper, Stronger, and Faster Multi-Person Pose Estimation Model. In ECCV'16, by Insafutdinov, E., Pishchulin, L., Andres, B., Andriluka, M., Schiele, B.
Understanding Collective Activities of People from Videos. In PAMI'14, by Choi, W., Savarese S.
Recovering 3D Human Body Configurations Using Shape Contexts. In PAMI'2006, by Mori, G., Malik, J.
Practical information
- General public
- Free
Contact
- EDIC - edic@epfl.ch