Gromov-Wasserstein in a Riemannian framework with applications to neuroimaging
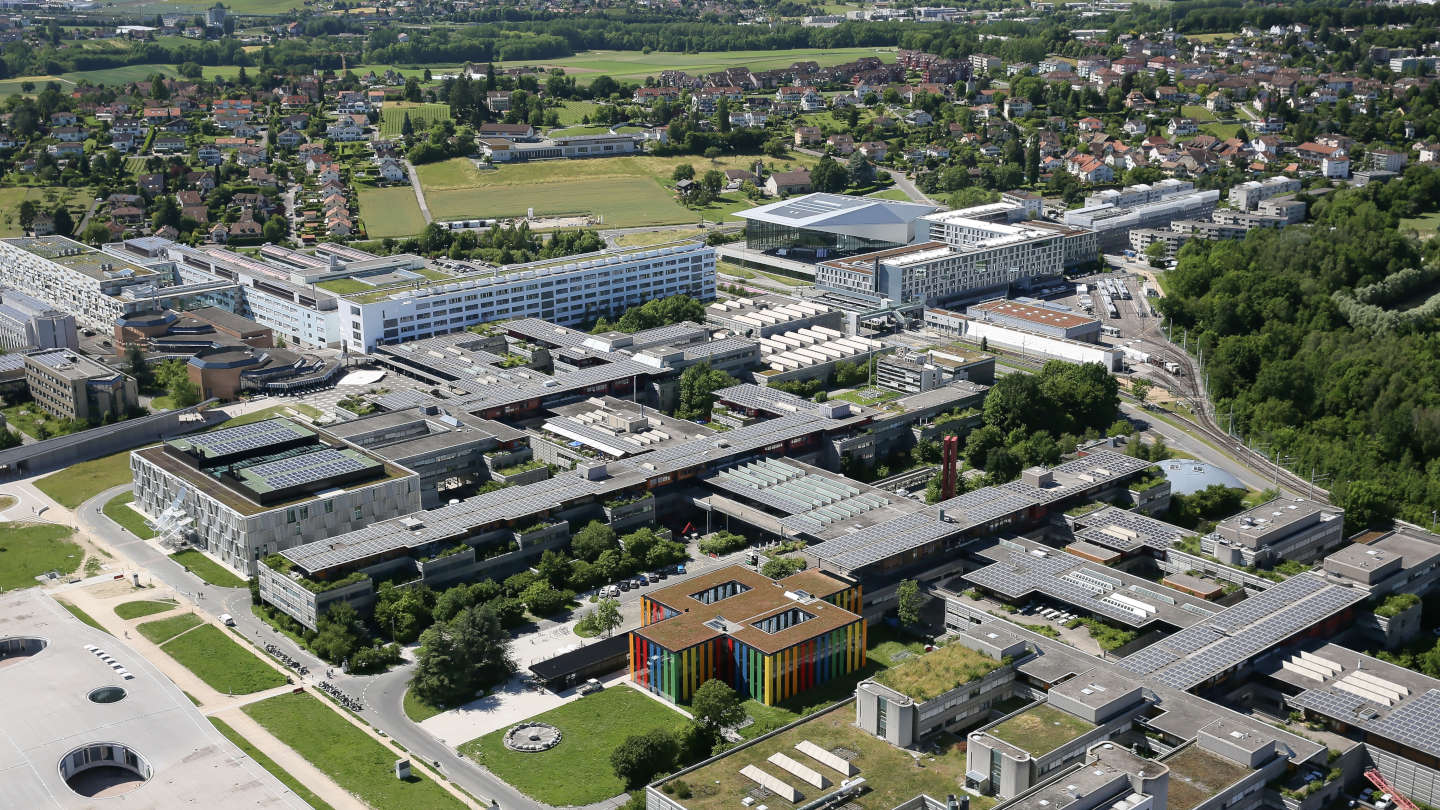
Event details
Date | 29.09.2020 |
Hour | 16:15 › 17:15 |
Speaker | Samir Chowdhury |
Category | Conferences - Seminars |
Applied Topology Seminar
Speaker: Samir Chowdhury, Stanford University
Title: Gromov-Wasserstein in a Riemannian framework with applications to neuroimaging
Abstract: Geometric and topological data analysis methods are increasingly being used in human neuroimaging studies to derive insights into neurobiology and behavior. We will begin by describing a pipeline that utilizes the Mapper algorithm to produce network representations of whole-brain activity during ongoing cognition. When applying this pipeline at scale across clinical populations, however, generating consistent insights requires the development of statistical learning techniques such as averaging and PCA across graphs without known node correspondences. We formulate this problem using the Gromov-Wasserstein (GW) distance and present a recently-developed Riemannian framework for GW-based graph averaging, partitioning, and tangent PCA. This framework permits using derived network representations beyond graph geodesic distances or adjacency matrices. In particular, we show that compared to state-of-the-art implementations that use adjacency matrix formulations, a spectral network representation leads to improved accuracy and runtime in graph learning tasks. Additionally, we observe that the spectral approach to GW graph partitioning corresponds to a generalization of Fiedler bipartitioning, thus suggesting new avenues for rigorous analysis of the GW problem.
More information on the website of the seminar: https://www.epfl.ch/labs/hessbellwald-lab/seminar/apptopsem2021/#chowdhury
If you wish to join the zoom meeting, please send an email to adelie.garin@epfl.ch or celia.hacker@epfl.ch
Speaker: Samir Chowdhury, Stanford University
Title: Gromov-Wasserstein in a Riemannian framework with applications to neuroimaging
Abstract: Geometric and topological data analysis methods are increasingly being used in human neuroimaging studies to derive insights into neurobiology and behavior. We will begin by describing a pipeline that utilizes the Mapper algorithm to produce network representations of whole-brain activity during ongoing cognition. When applying this pipeline at scale across clinical populations, however, generating consistent insights requires the development of statistical learning techniques such as averaging and PCA across graphs without known node correspondences. We formulate this problem using the Gromov-Wasserstein (GW) distance and present a recently-developed Riemannian framework for GW-based graph averaging, partitioning, and tangent PCA. This framework permits using derived network representations beyond graph geodesic distances or adjacency matrices. In particular, we show that compared to state-of-the-art implementations that use adjacency matrix formulations, a spectral network representation leads to improved accuracy and runtime in graph learning tasks. Additionally, we observe that the spectral approach to GW graph partitioning corresponds to a generalization of Fiedler bipartitioning, thus suggesting new avenues for rigorous analysis of the GW problem.
More information on the website of the seminar: https://www.epfl.ch/labs/hessbellwald-lab/seminar/apptopsem2021/#chowdhury
If you wish to join the zoom meeting, please send an email to adelie.garin@epfl.ch or celia.hacker@epfl.ch
Links
Practical information
- Informed public
- Free
Organizer
- Applied Topology Seminar