Minimum Description Length Interpolation Learning
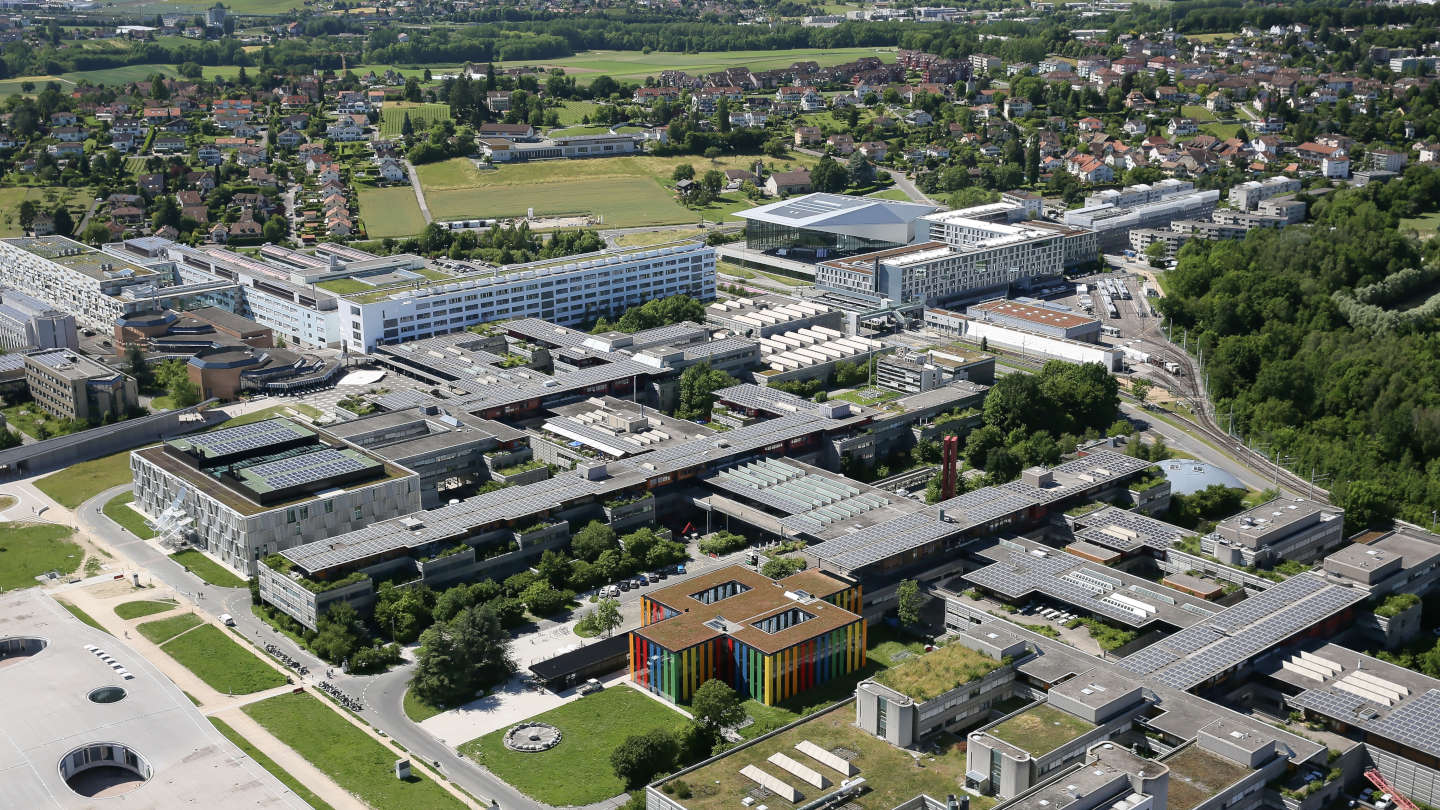
Event details
Date | 09.06.2023 |
Hour | 11:15 › 12:15 |
Speaker | Nati Srebro (TTI Chicago) |
Location | |
Category | Conferences - Seminars |
Event Language | English |
We consider learning by using the shortest program that perfectly fits the training data, even in situations where labels are noisy and there is no way of exactly predicting the labels on the population. Classical theory tells us that in such situations we should balance program length with training error, in which case we can compete with any (unknown) program with sample complexity proportional to the length of the program. But in the spirit of recent work on benign overfitting, we ignore this wisdom and insist on zero training error even in noisy situations. We study the generalization property of the shortest program interpolator, and ask how it performs compared to the balanced approach, and how much we suffer, if at all, due to such overfitting.
Practical information
- Expert
- Free
Organizer
- Chizat Lénaïc Ged François