Reinforcement Learning and Model Predictive Control, where are we?
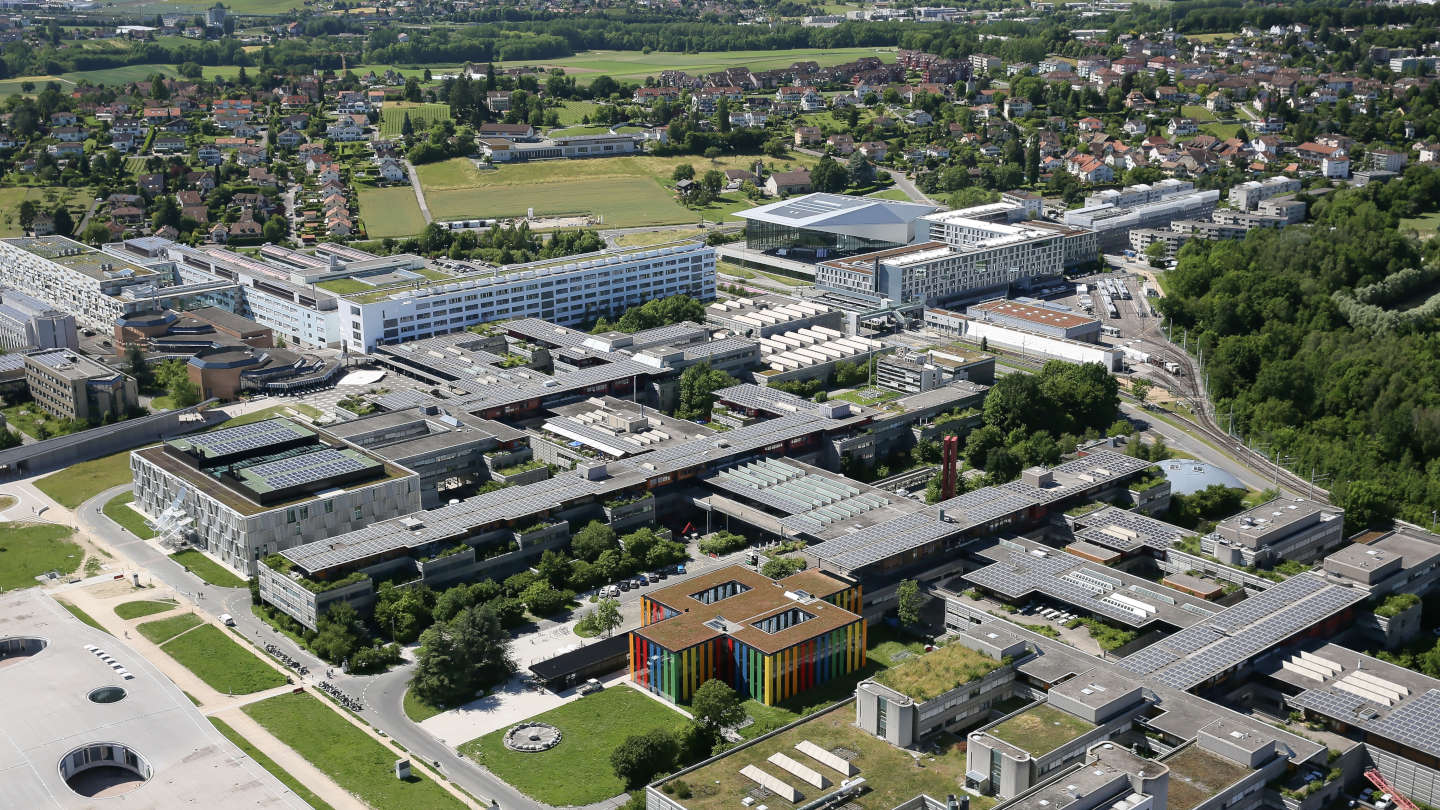
Event details
Date | 19.09.2023 |
Hour | 14:00 › 15:00 |
Speaker | Sébastien Gros (NTNU, Trondheim, Norway) |
Location | |
Category | Conferences - Seminars |
Abstract:
The use of data-driven methods in MPC has been drawing an important interest in research over the past few years. Different techniques are being advocated, often arguing for using tools from Machine Learning in the MPC model, or even for using completely model-free approaches. The hope, arguably, is that improving the MPC predictions results in increased closed-loop performance. However, recent results show that when that performance is assessed in economic terms (energy, emission, production, time, money), the role of the MPC model is less obvious than usually assumed. In this talk, we will unpack this question. We will see how an MPC scheme is in fact a model of the solution of a Markov Decision Process as a whole, rather than a control tool built around a prediction model. This observation will allow us to connect MPC to Reinforcement Learning (RL), and understand how to use RL techniques to improve the MPC closed-loop performance directly. Finally, this observation will also provide insights as to when MPC built with classic data-driven modelling techniques can be expected to produce good closed-loop performance.
Bio:
Sebastien Gros received his Ph.D degree from EPFL, Switzerland, in 2007. After a journey by bicycle from Switzerland to the Everest base camp in full autonomy, he joined a R&D group hosted at Strathclyde University focusing on wind turbine control. In 2011, he joined the university of KU Leuven as a postdoc, where his main research focus was on optimal control and fast MPC for complex mechanical systems. He joined the Department of Signals and Systems at Chalmers University of Technology, Göteborg in 2013, where he became associate Prof. in 2017. He is now full Prof. and Head of Dept. of Cybernetics at NTNU, Norway and guest Prof. at Chalmers. His main research interests include MPC, Markov Decision Processes, Learning for MPC, Economic MPC, stochastic optimal control, Reinforcement Learning, numerical methods, and energy-related applications.
Practical information
- General public
- Free