Reinforcement Learning for Optimal Control with Stability Guarantees
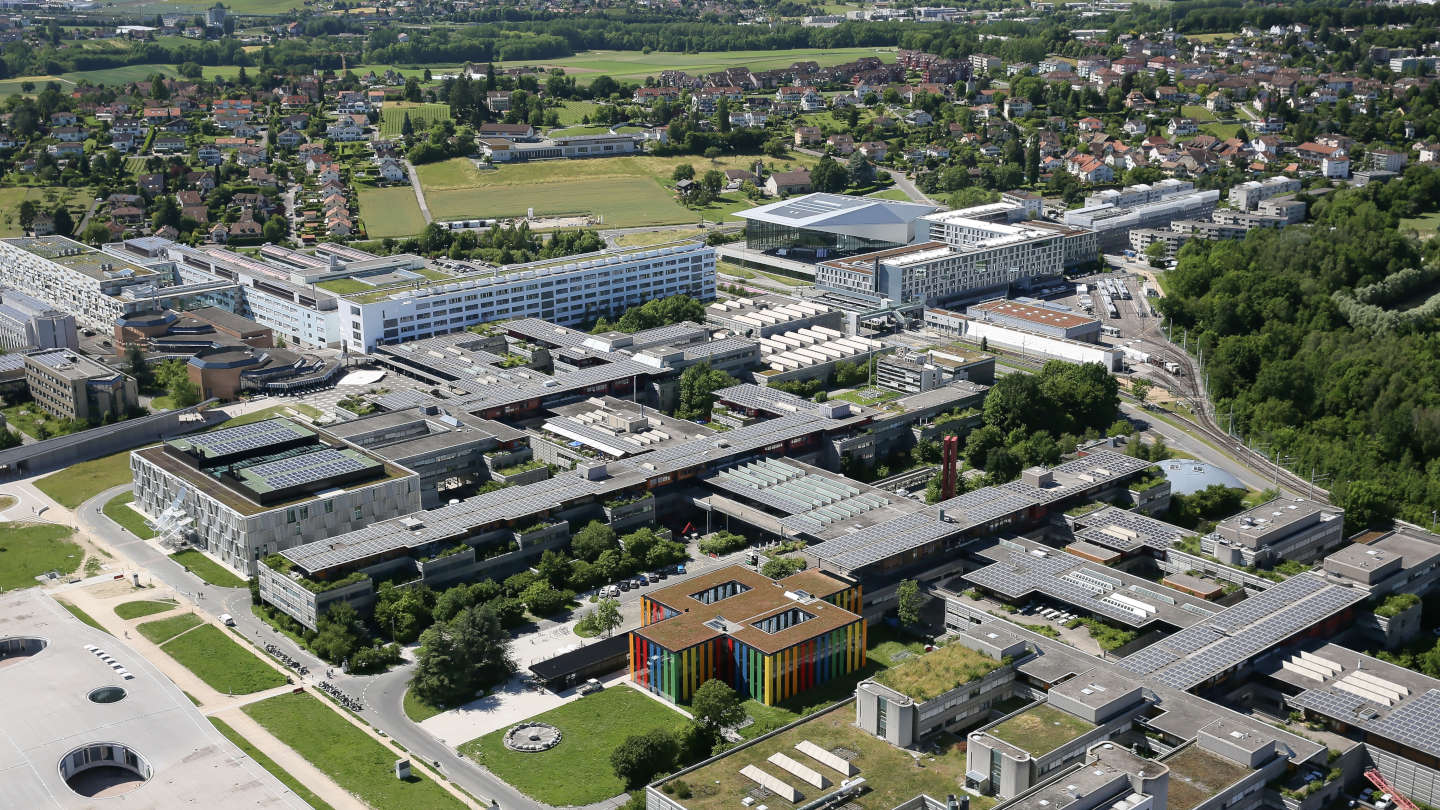
Event details
Date | 14.04.2025 |
Hour | 14:00 › 15:00 |
Speaker | Sucheth Shenoy, Master Student Hamburg University of Technology (TUHH) |
Location | |
Category | Conferences - Seminars |
Event Language | English |
Abstract:
As reinforcement learning (RL) gains traction in control applications, a central challenge remains: ensuring closed-loop stability throughout training and deployment. This talk introduces Magnitude and Direction (MAD) policies, a novel policy parameterization that enables RL with formal stability guarantees for pre-stabilized nonlinear systems. MAD policies decompose the control signal into two components: a direction term, parameterized by expressive function approximators (e.g., neural networks), and a magnitude term, constrained by ℓp-stable disturbance-feedback operators. This structure preserves the flexibility of modern RL methods while guaranteeing ℓp closed-loop stability.
MAD policies extend classical disturbance-feedback (DF) approaches, such as those in the Performance-Boosting framework, by explicitly incorporating state-feedback—enhancing learning efficiency and generalization in model-free settings without compromising safety. The talk will cover theoretical guarantees, robustness under model mismatch, and practical implementation with model-free actor-critic algorithms like DDPG. We demonstrate the effectiveness of the MAD policy on a benchmark corridor-navigation task, where it matches the performance and generalization of standard neural policies, while ensuring stability by design. Future research directions include testing and adapting the MAD policy class in more complex environments.
Biosketch:
Sucheth Shenoy is a Master’s student in Mechatronics with a specialization in Robotics and Intelligent Systems at Hamburg University of Technology (TUHH). He holds a Bachelor’s degree in Mechanical Engineering from RV College of Engineering (RVCE), Bengaluru, India. He is currently completing his Master’s thesis on Reinforcement Learning for Optimal Control with Stability Guarantees at the DECODE group, Automatic Control Laboratory, EPFL, under the supervision of Dr. Luca Furieri, Dr. Danilo Saccani, and Prof. Giancarlo Ferrari-Trecate. His work is supported by the NCCR Automation Fellowship. his research interests lie at the intersection of learning-based control and robotics.
Practical information
- General public
- Free
Organizer
- Professor Giancarlo Ferrari Trecate
Contact
- barbara.schenkel@epfl.ch chantal.demont@epfl.ch