Spatially heterogeneous learning in a deep neural network
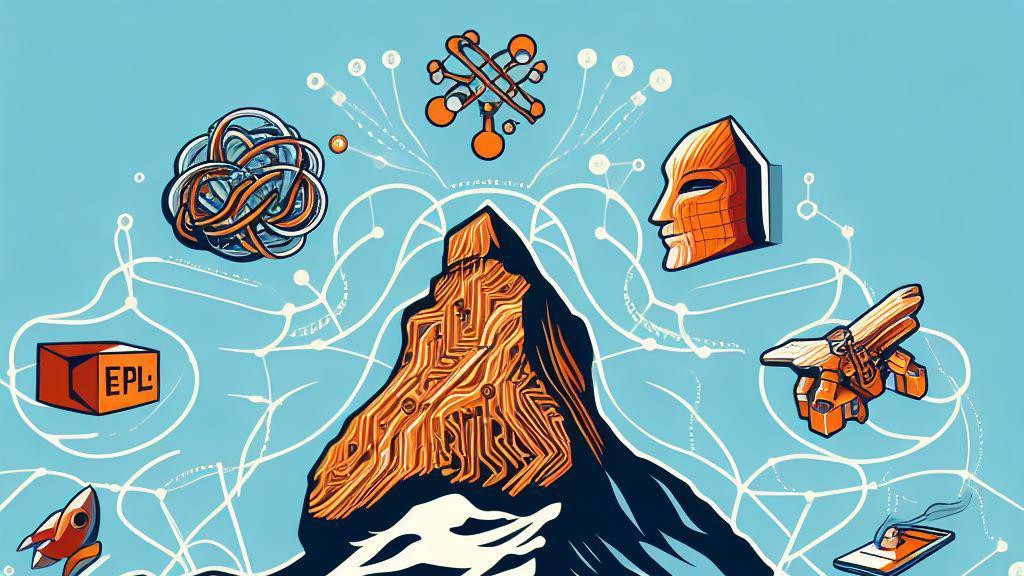
Event details
Date | 15.05.2024 |
Hour | 11:15 › 12:15 |
Speaker | Hajime Yoshino |
Location | |
Category | Conferences - Seminars |
Event Language | English |
In this talk I discuss statistical mechanical properties of typical machines in an ensemble of multi-layer perceptrons of width N and depth L which are performing exactly the same learning task independently from each other [1] following the line of research started by E. Gardner in 1980s. The natural order parameters associated with the ensemble are the overlaps that measure similarity between the configurations of the machines in the hidden layers. By theoretical and numerical analysis we found that the order parameters evolve in space, along the axis perpendicular to the layers. Typically we find that the order parameters become smaller in the center of the network suggesting that the system is more constrained close to the input/output boundaries while less constrained in the center. The situation is reminiscent of the wetting transitions switched on by 'walls' found in physical systems [2]
and the spatially coupled inference problems [3]. On the theoretical side, we developed a replica method to analyze the two canonical learning scenarios
used often in statistical mechanics of machine learning, namely random scenario and Bayes-optimal teacher-student scenario. We found not only the amplitude of the order parameters but also the hierarchy of replica symmetry breaking (when it happens) evolves in space. On the numerical side, we performed simulations analyzing the two learning scenarios as in the theory and also analyzed realistic learning tasks on MNIST data. Numerical simulations suggest that the dynamics (MC or SGD) eventually bring the system to thermal equilibrium characterized by space-dependent overlaps.
[1] H. Yoshino, SciPost Physics Core 2,005 (2020) and Phys. Rev. Research 5, 033068 (2023).
[2] F. Krzakala and L. Zdeborová, J. Chem. Phys. 134, 034512 (2011) and J. Chem. Phys. 134, 034513 (2011).
[3] L. Zdeborová and F. Krzakala, Advances in Physic 65, 453 (2016).
Practical information
- Informed public
- Free
Contact
- lenaic.chizat@epfl.ch