Talk of Professor Taiji Suzuki (University of Tokyo)
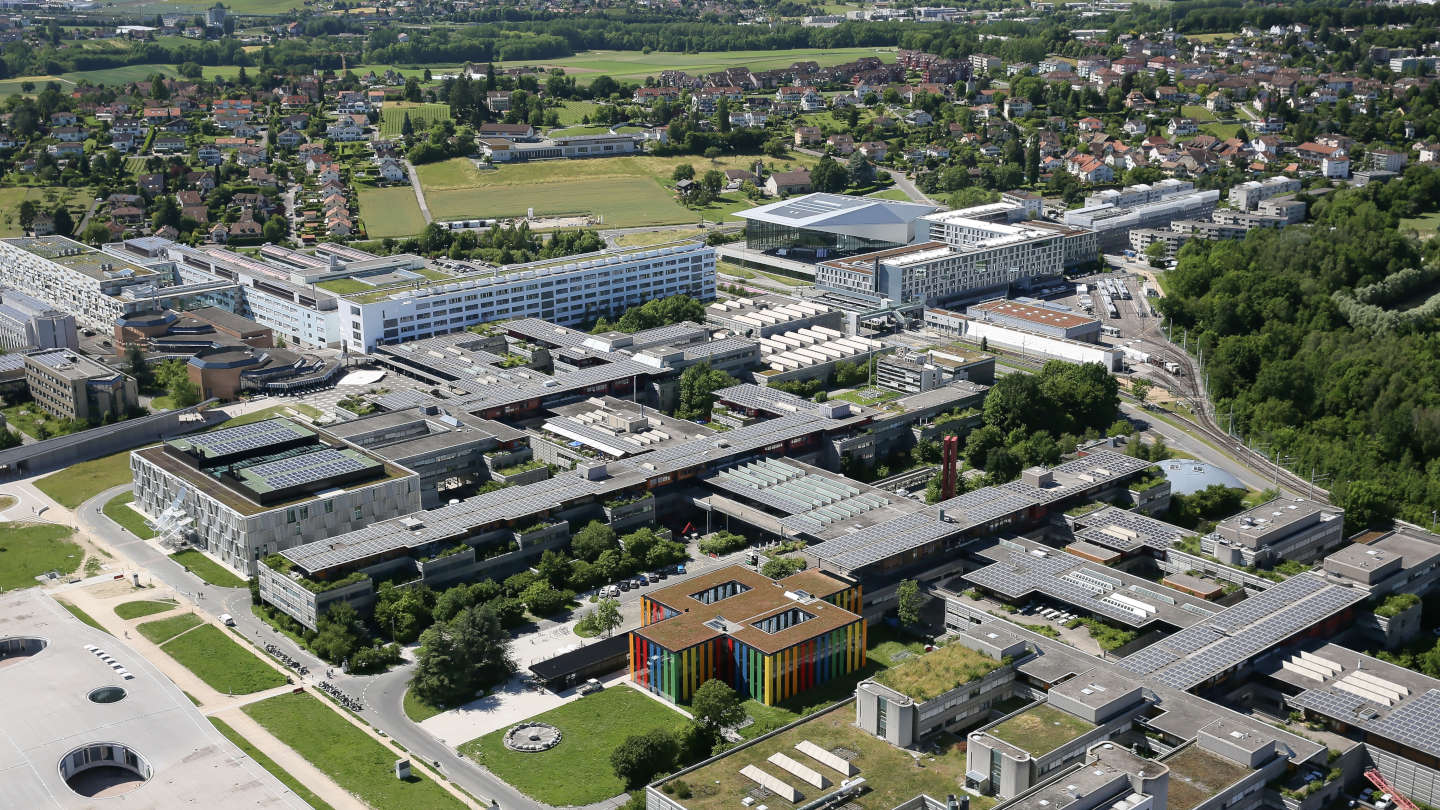
Event details
Date | 01.11.2019 |
Hour | 12:00 › 14:00 |
Speaker | Professor Taiji Suzuki |
Location | |
Category | Conferences - Seminars |
Title:
Generalization analysis and optimization of deep learning: adaptivity and kernel view
Abstract: In this talk, I will discuss the adaptivity of deep learning, and the generalization ability and optimization property under overparameterized settings. In the first half, we theoretically show that deep learning can extract appropriate bases in an adaptive way and thus can achieve superior performance than kernel methods especially on models with non-convexity property. Thanks to this properties, deep learning can outperform kernel methods if input data are high dimensional and the target functions are in Besov space.
In the later half, we discuss the generalization ability and optimization property of deep learning under overparameterized settings. The classical learning theory suggests that overparameterized models cause overfitting. However, practically used large deep models avoid overfitting, which is not well explained by the classical approaches. To resolve this issue, we give a new unified frame-work for deriving a compression based bound. The existing compression based bounds can only be applied to a compressed network, but our bound can convert those bounds to that of non-compressed original network. Finally, we discuss the optimization aspects of neural networks under the neural tangent kernel regime. We show that for a classification task, the width of networks can be much smaller than existing studies to obtain a near global optimal solution by a gradient descent.
BIO: Taiji Suzuki is currently an Associate Professor in the Department of Mathematical Informatics at the University of Tokyo. He also serves as the team leader of "deep learning theory group" in AIP-RIKEN. He received his Ph.D. degree in information science and technology from the University of Tokyo in 2009. He has a broad research interest in statistical learning theory on deep learning, kernel methods and sparse estimation, and stochastic optimization for large-scale machine learning problems. He served as technical program committee members of premier conferences such as NeurIPS, ICML, ICLR, COLT, AISTATS and ACML. He received Outstanding Achievement Award in 2017 from the Japan Statistical Society, Outstanding Achievement Award in 2016 from the Japan Society for Industrial and Applied Mathematics, and Best Paper Award in 2012 from IBISML.
Generalization analysis and optimization of deep learning: adaptivity and kernel view
Abstract: In this talk, I will discuss the adaptivity of deep learning, and the generalization ability and optimization property under overparameterized settings. In the first half, we theoretically show that deep learning can extract appropriate bases in an adaptive way and thus can achieve superior performance than kernel methods especially on models with non-convexity property. Thanks to this properties, deep learning can outperform kernel methods if input data are high dimensional and the target functions are in Besov space.
In the later half, we discuss the generalization ability and optimization property of deep learning under overparameterized settings. The classical learning theory suggests that overparameterized models cause overfitting. However, practically used large deep models avoid overfitting, which is not well explained by the classical approaches. To resolve this issue, we give a new unified frame-work for deriving a compression based bound. The existing compression based bounds can only be applied to a compressed network, but our bound can convert those bounds to that of non-compressed original network. Finally, we discuss the optimization aspects of neural networks under the neural tangent kernel regime. We show that for a classification task, the width of networks can be much smaller than existing studies to obtain a near global optimal solution by a gradient descent.
BIO: Taiji Suzuki is currently an Associate Professor in the Department of Mathematical Informatics at the University of Tokyo. He also serves as the team leader of "deep learning theory group" in AIP-RIKEN. He received his Ph.D. degree in information science and technology from the University of Tokyo in 2009. He has a broad research interest in statistical learning theory on deep learning, kernel methods and sparse estimation, and stochastic optimization for large-scale machine learning problems. He served as technical program committee members of premier conferences such as NeurIPS, ICML, ICLR, COLT, AISTATS and ACML. He received Outstanding Achievement Award in 2017 from the Japan Statistical Society, Outstanding Achievement Award in 2016 from the Japan Society for Industrial and Applied Mathematics, and Best Paper Award in 2012 from IBISML.
Practical information
- Expert
- Registration required
- This event is internal
Organizer
- Professor Volkan Cevher
Contact
- Gosia Baltaian