Design tradeoffs of approximate analog neural accelerators
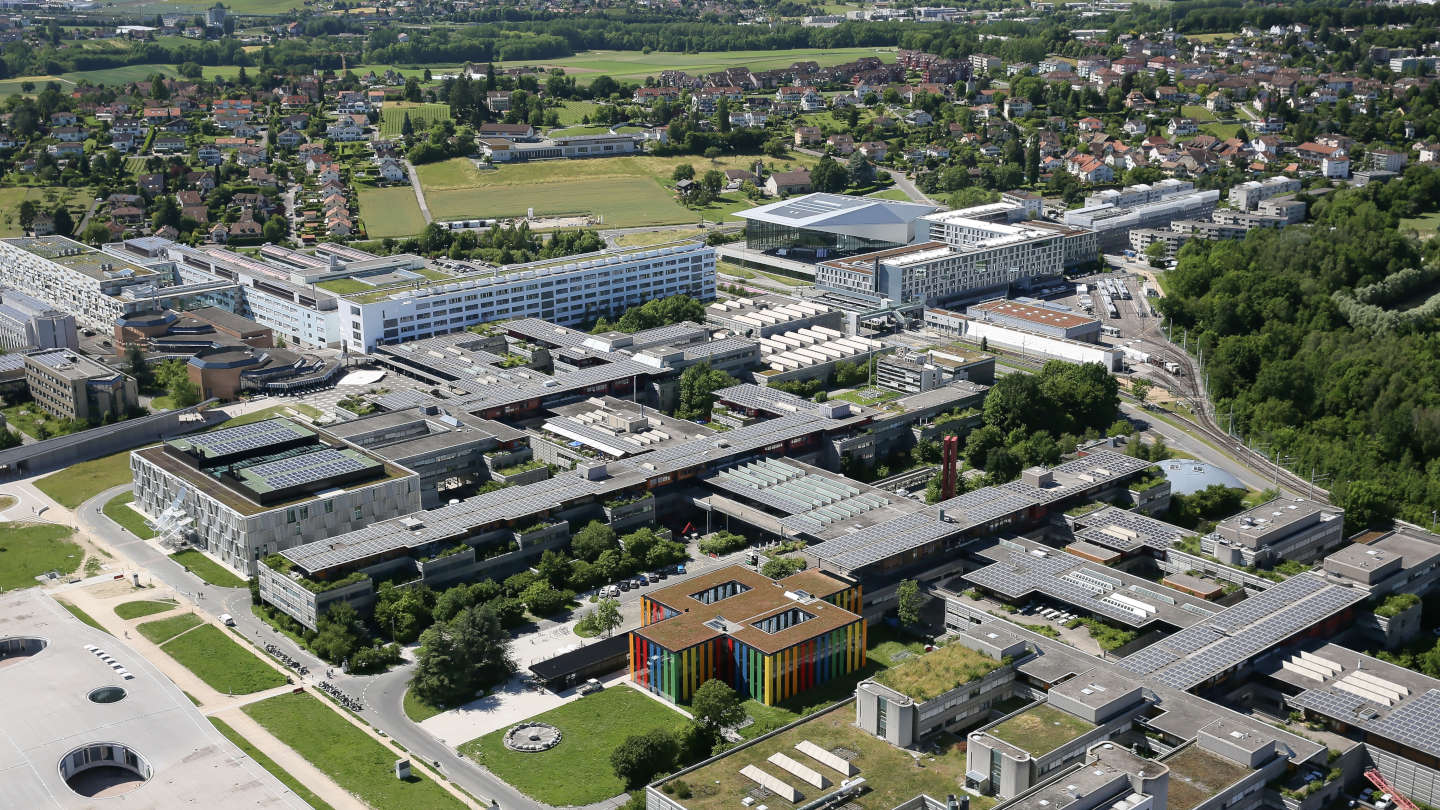
Event details
Date | 25.01.2013 |
Hour | 12:30 › 14:00 |
Speaker | Renée St.Amant |
Location | |
Category | Conferences - Seminars |
Abstract:
An increased focus on energy efficiency, coupled with increasingly less reliable hardware behavior due to transistor scaling, has motivated research in the use of approximate computing to gain efficiency when precise operation is not required. Prior work has proposed an approach to translate general-purpose, approximation-tolerant program segments to neural networks for acceleration, exploiting opportunities for energy efficiency through techniques that compromise accuracy. As analog circuits traditionally trade accuracy for efficiency, and therefore exhibit high potential, we explore their application to a neural accelerator in the context of enabling the efficient acceleration of general-purpose, approximate code. We discuss the opportunities, challenges, and tradeoffs that are unique to an analog approach, while defining two major design components to consider: value representation, including bit-width selection, and compute unit configuration.
Bio:
Renée St. Amant is a Ph.D. candidate in the Computer Sciences Department at the University of Texas at Austin, working under the supervision of Doug Burger. Her research interests are in the area of computer architecture and include mixed-signal microarchitecture design, approximate computing, and memory technologies. Her current work aims to enable emerging applications through mixed-signal design for energy-efficient, approximate computation.
An increased focus on energy efficiency, coupled with increasingly less reliable hardware behavior due to transistor scaling, has motivated research in the use of approximate computing to gain efficiency when precise operation is not required. Prior work has proposed an approach to translate general-purpose, approximation-tolerant program segments to neural networks for acceleration, exploiting opportunities for energy efficiency through techniques that compromise accuracy. As analog circuits traditionally trade accuracy for efficiency, and therefore exhibit high potential, we explore their application to a neural accelerator in the context of enabling the efficient acceleration of general-purpose, approximate code. We discuss the opportunities, challenges, and tradeoffs that are unique to an analog approach, while defining two major design components to consider: value representation, including bit-width selection, and compute unit configuration.
Bio:
Renée St. Amant is a Ph.D. candidate in the Computer Sciences Department at the University of Texas at Austin, working under the supervision of Doug Burger. Her research interests are in the area of computer architecture and include mixed-signal microarchitecture design, approximate computing, and memory technologies. Her current work aims to enable emerging applications through mixed-signal design for energy-efficient, approximate computation.
Practical information
- Informed public
- Free
- This event is internal
Organizer
- Dr Boris Grot, PARSA
Contact
- Stéphanie Baillargues