A New Geometric Approach to Topic Modeling and Discovery
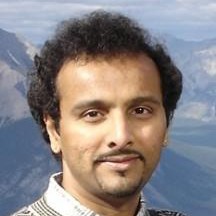
Event details
Date | 27.11.2013 |
Hour | 16:15 › 17:15 |
Speaker | Prof. Prakash Ishwar, Boston University |
Location | |
Category | Conferences - Seminars |
In this talk I will present a new algorithm for topic discovery based
on the geometry of cross-document word-frequency patterns. The
geometric perspective gains significance under the so called
separability condition that posits the existence of novel-words that
are unique to each topic. The algorithm utilizes random projections to
identify novel words and associated topics. The key insight here is
that the maximum and minimum values of cross-document frequency
patterns projected along any direction are associated with novel
words. In contrast to ML and Bayesian approaches that require solving
non-convex optimization problems using approximations or heuristics,
the new algorithm is convex, asymptotically consistent, and has
provable performance guarantees. While our sample complexity bounds
for topic recovery are similar to the state-of-art, the computational
complexity of our scheme scales linearly with the number of documents
and the number of words per document. We present several experiments
on synthetic and realworld datasets to demonstrate qualitative and
quantitative merits of our scheme. This talk is based on joint work
with Ding, Rohban, and Saligrama at Boston University.
on the geometry of cross-document word-frequency patterns. The
geometric perspective gains significance under the so called
separability condition that posits the existence of novel-words that
are unique to each topic. The algorithm utilizes random projections to
identify novel words and associated topics. The key insight here is
that the maximum and minimum values of cross-document frequency
patterns projected along any direction are associated with novel
words. In contrast to ML and Bayesian approaches that require solving
non-convex optimization problems using approximations or heuristics,
the new algorithm is convex, asymptotically consistent, and has
provable performance guarantees. While our sample complexity bounds
for topic recovery are similar to the state-of-art, the computational
complexity of our scheme scales linearly with the number of documents
and the number of words per document. We present several experiments
on synthetic and realworld datasets to demonstrate qualitative and
quantitative merits of our scheme. This talk is based on joint work
with Ding, Rohban, and Saligrama at Boston University.
Practical information
- Informed public
- Free
Organizer
- IPG Seminar - muriel.bardet@epfl.ch
Contact
- Host: Prof. Michael Gastpar - LINX