Algorithms for Constrained Determinantal Point Processes using Mixed Discriminants
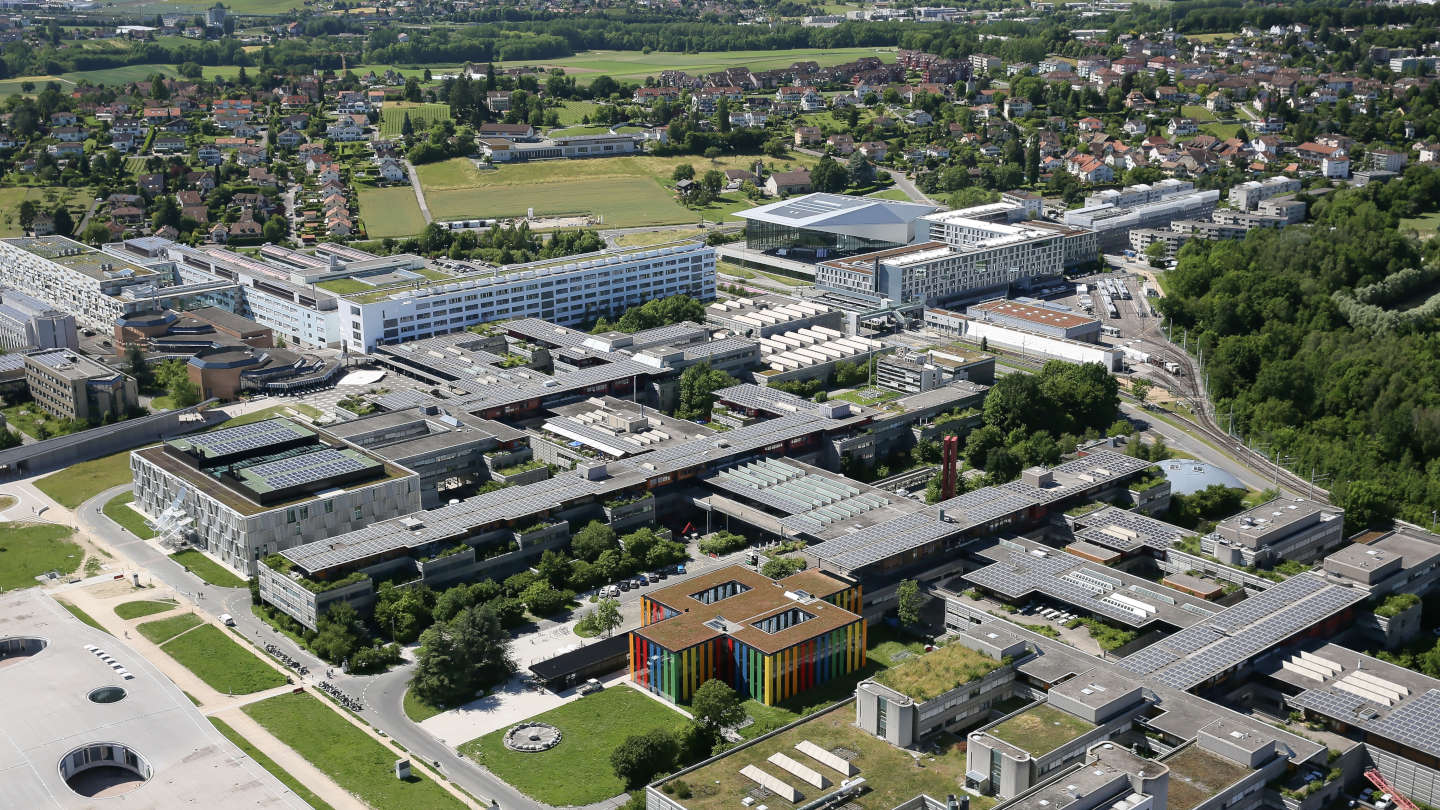
Event details
Date | 24.07.2018 |
Hour | 12:15 › 14:15 |
Speaker | Vijay Keswani |
Location | |
Category | Conferences - Seminars |
EDIC candidacy exam
Exam president: Prof. Patrick Thiran
Thesis advisor: Prof. Nisheeth Vishnoi
Co-examiner: Dr. Olivier Levêque
Abstract
Determinantal Point Processes (DPPs) are probability distributions defined over subsets of a ground set of elements, with the property that the subsets that are more âdiverseâ have higher probability. They occur in many areas of theoretical computer science, machine learning, optimization and physics, and for certain simple settings, are known to have efficient algorithms. Anari, Gharan and Rezaei (2016) gave one such Monte Carlo Markov Chain algorithm for sampling from Strongly Rayleigh distributions, a generalisation of DPPs, when there is a simple cardinality constraint on the elements of the support of the DPP distribution. However, when more complex constraints are considered, the problem becomes intractable. Celis et. al (2017) gave a polynomial time algorithm for sampling from DPPs with partition constraints when the description of the constraints is in unary, but also showed that when the description is in binary, sampling from such DPPs is #P-hard and reduces to computing mixed discriminants. For special cases though, mixed discriminants can be approximated within a polynomial factor, as shown by Barvinok (2015) in the case of well-conditioned matrix tuples, and this result can potentially be used to derive polynomial-approximation sampling algorithms for certain settings of constrained DPPs. The algorithms for sampling from DPPs with partition constraints can also potentially be extended to partition-group constraints, where there can be non-empty overlap between the various divisions of the ground set. Such constraints more accurately depict the real-world data, where each individual can belong to multiple groups, for example, by gender and race.
Background papers
Monte Carlo Markov Chain Algorithms for Sampling Strongly Rayleigh Distributions and Determinantal Point Processes
On the Complexity of Constrained Determinantal Point Processes
Concentration of the mixed discriminant of well-conditioned matrices
Exam president: Prof. Patrick Thiran
Thesis advisor: Prof. Nisheeth Vishnoi
Co-examiner: Dr. Olivier Levêque
Abstract
Determinantal Point Processes (DPPs) are probability distributions defined over subsets of a ground set of elements, with the property that the subsets that are more âdiverseâ have higher probability. They occur in many areas of theoretical computer science, machine learning, optimization and physics, and for certain simple settings, are known to have efficient algorithms. Anari, Gharan and Rezaei (2016) gave one such Monte Carlo Markov Chain algorithm for sampling from Strongly Rayleigh distributions, a generalisation of DPPs, when there is a simple cardinality constraint on the elements of the support of the DPP distribution. However, when more complex constraints are considered, the problem becomes intractable. Celis et. al (2017) gave a polynomial time algorithm for sampling from DPPs with partition constraints when the description of the constraints is in unary, but also showed that when the description is in binary, sampling from such DPPs is #P-hard and reduces to computing mixed discriminants. For special cases though, mixed discriminants can be approximated within a polynomial factor, as shown by Barvinok (2015) in the case of well-conditioned matrix tuples, and this result can potentially be used to derive polynomial-approximation sampling algorithms for certain settings of constrained DPPs. The algorithms for sampling from DPPs with partition constraints can also potentially be extended to partition-group constraints, where there can be non-empty overlap between the various divisions of the ground set. Such constraints more accurately depict the real-world data, where each individual can belong to multiple groups, for example, by gender and race.
Background papers
Monte Carlo Markov Chain Algorithms for Sampling Strongly Rayleigh Distributions and Determinantal Point Processes
On the Complexity of Constrained Determinantal Point Processes
Concentration of the mixed discriminant of well-conditioned matrices
Practical information
- General public
- Free
Contact
- EDIC - edic@epfl.ch