Autoformalization: from traditional to formal mathematics using large language models
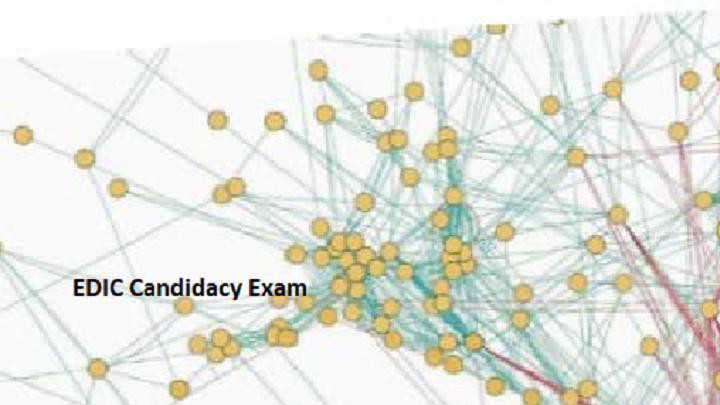
Event details
Date | 17.06.2024 |
Hour | 15:00 › 17:00 |
Speaker | Auguste Poiroux |
Location | |
Category | Conferences - Seminars |
EDIC candidacy exam
Exam president: Prof. Caglar Gulcehre
Thesis advisor: Prof. Viktor Kuncak
Thesis co-advisor: Prof. Antoine Bosselut
Co-examiner: Prof. Emmanuel Abbé
Abstract
In the past decade, advancements in natural language processing (NLP) have been transformative. Modern large language models (LLMs) facilitate nuanced conversations and demonstrate potential in tool manipulation and reasoning. These developments invite investigations into their applications across various fields, including mathematics.
Despite significant progress, LLMs continue to face substantial challenges in handling complex, extended reasoning sequences. Traditional methods of manually verifying mathematical reasoning are not only labor-intensive but also require expertise. A promising alternative involves the use of formal languages. By constraining LLMs to generate structured formal languages, we can automate the verification of reasoning processes, eliminating the need for continuous human oversight. A closely related task, known as autoformalization, involves training models to translate natural language into formal language. Such capabilities could enable LLMs to independently verify their reasoning, translate and validate extensive traditional mathematical corpora into formal systems, and unlock several orders of magnitudes of training data for automated theorem provers. This candidacy exam explores the potential and current boundaries of autoformalization.
Background papers
- Autoformalization with Large Language Models
link: https://arxiv.org/abs/2205.12615
- ProofNet: Autoformalizing and Formally Proving Undergraduate-Level Mathematics
link: https://arxiv.org/abs/2302.12433
- Draft, Sketch, and Prove: Guiding Formal Theorem Provers with Informal Proofs
link: https://arxiv.org/abs/2210.12283
Exam president: Prof. Caglar Gulcehre
Thesis advisor: Prof. Viktor Kuncak
Thesis co-advisor: Prof. Antoine Bosselut
Co-examiner: Prof. Emmanuel Abbé
Abstract
In the past decade, advancements in natural language processing (NLP) have been transformative. Modern large language models (LLMs) facilitate nuanced conversations and demonstrate potential in tool manipulation and reasoning. These developments invite investigations into their applications across various fields, including mathematics.
Despite significant progress, LLMs continue to face substantial challenges in handling complex, extended reasoning sequences. Traditional methods of manually verifying mathematical reasoning are not only labor-intensive but also require expertise. A promising alternative involves the use of formal languages. By constraining LLMs to generate structured formal languages, we can automate the verification of reasoning processes, eliminating the need for continuous human oversight. A closely related task, known as autoformalization, involves training models to translate natural language into formal language. Such capabilities could enable LLMs to independently verify their reasoning, translate and validate extensive traditional mathematical corpora into formal systems, and unlock several orders of magnitudes of training data for automated theorem provers. This candidacy exam explores the potential and current boundaries of autoformalization.
Background papers
- Autoformalization with Large Language Models
link: https://arxiv.org/abs/2205.12615
- ProofNet: Autoformalizing and Formally Proving Undergraduate-Level Mathematics
link: https://arxiv.org/abs/2302.12433
- Draft, Sketch, and Prove: Guiding Formal Theorem Provers with Informal Proofs
link: https://arxiv.org/abs/2210.12283
Practical information
- General public
- Free
Contact
- edic@epfl.ch