Constructive Initialization for Sigmoidal Neural Networks in Engineering Mechanics Applications
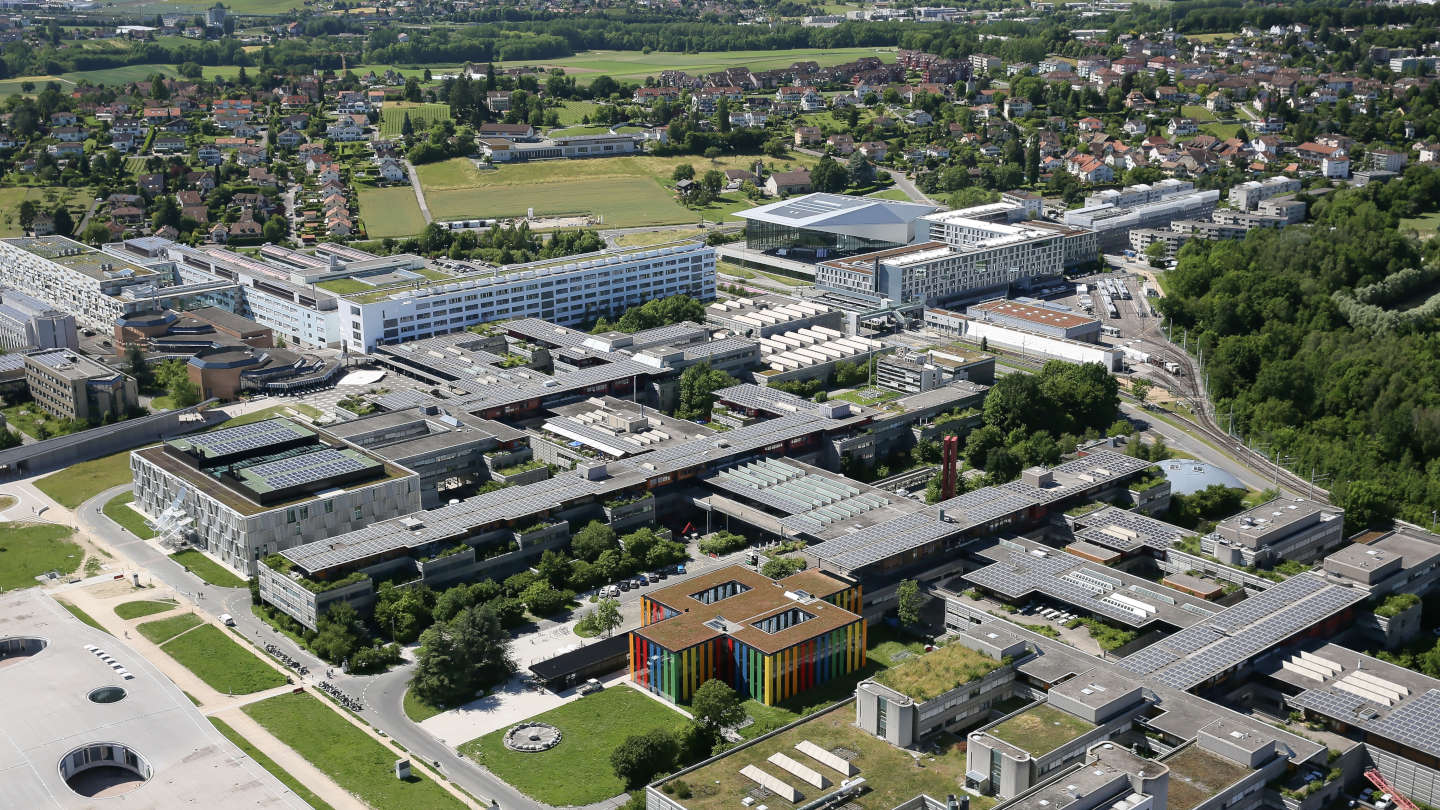
Event details
Date | 15.05.2018 |
Hour | 11:15 › 12:15 |
Speaker | Prof. Jin-Song Pei School of Civil Engineering and Environmental Science University of Oklahoma, Norman, Oklahoma, USA |
Location | |
Category | Conferences - Seminars |
Following recent advances in sensing and testing technologies, there is a pressing issue of handling experimental data, the quantity and quality of which have been significantly expanded. Facing mountains of data (with numerous unknowns and uncertainties), we need to extract the most useful and accurate underlying information for rapid assessment and critical decision making. Artificial neural networks (ANNs) and other nonparametric models provide alternatives when model-based methods or fixed basis functions are not effective. In engineering mechanics community, developing comprehensive and high-fidelity models for nonlinear dynamical systems has been one of the key research issues, impacting a very broad range of applications. If designed properly, ANNs are very suitable to process and analyze datasets collected from complex nonlinear dynamical systems and in a computationally efficient manner. The use of neural networks, however, has been a somewhat controversial subject. When the inner workings of ANNs are not transparent to most users, neural networks remain powerful but mysterious “black boxes”.
To demystify the reputed black-box, the research objective is to develop an insightful process of designing sigmoidal neural network architectures, which includes making choice of initial values of weights and biases and the number of hidden nodes, with a final goal of having good generalization capability of trained neural networks. Our research has developed direct (non-iterative) methods for: (a) utilizing a few basic “building blocks” (called “neural network prototypes”) individually or combinatorially and following a handful of proposed rules/guidelines to generate neural network architectures, and (b) selecting initial weights and biases. Thereby we have approximated (1) the four basic arithmetic operations, (2) polynomials, (3) the exponential function, (4) Gaussian and Mexican hat functions, (5) certain hardening and softening types of nonlinearities, and others. Our methodology and techniques have been validated using both experimental and simulated data.
Practical information
- General public
- Free
Organizer
- IMAC
Contact
- Sai G.S. Pai