Deep Structured Representation Learning for Visual Recognition
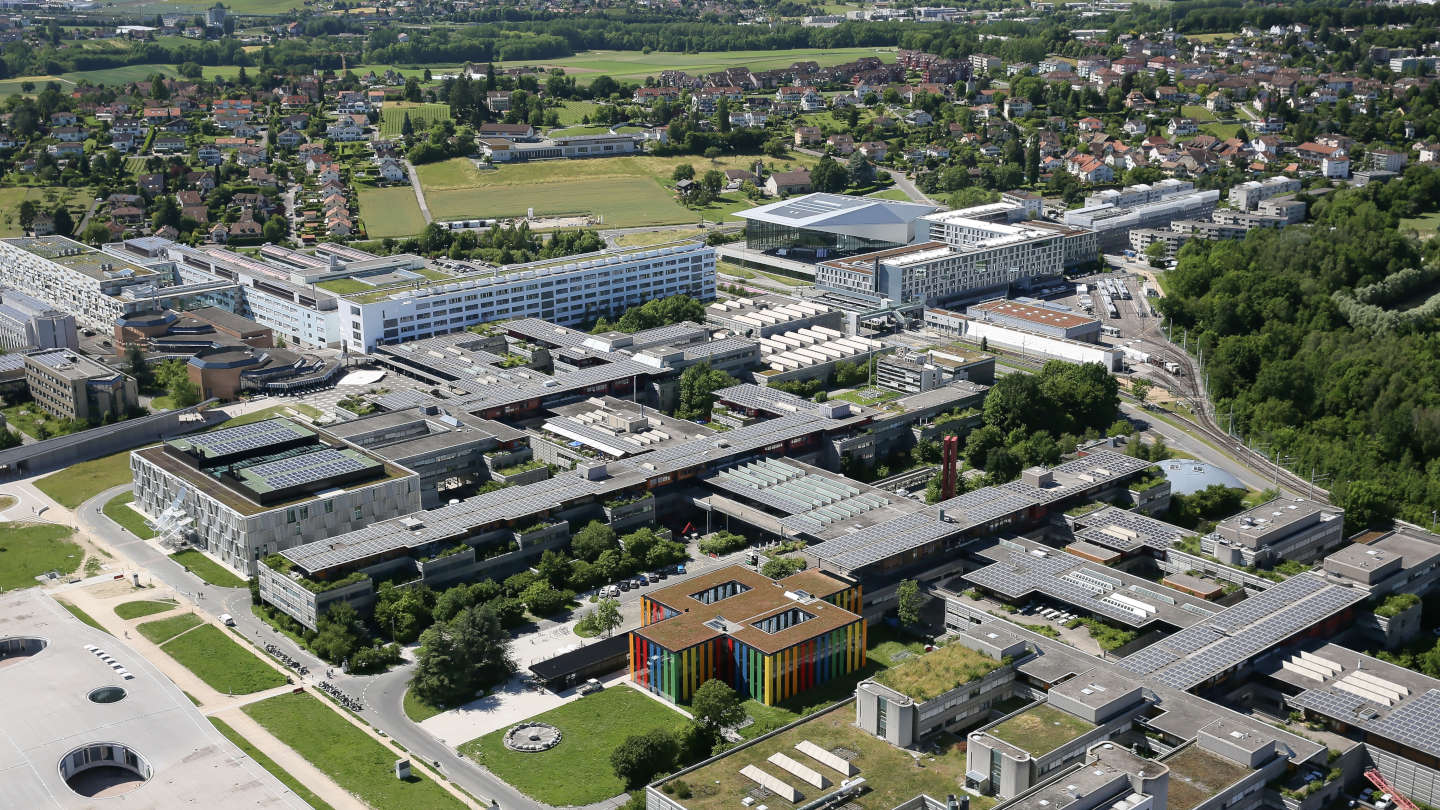
Event details
Date | 03.07.2018 |
Hour | 10:00 › 12:00 |
Speaker | Krishna Kanth Nakka |
Location | |
Category | Conferences - Seminars |
EDIC candidacy exam
Exam president: Prof. Sabine Süsstrunk
Thesis advisor: Prof. Pascal Fua
Thesis co-advisor: Dr Matthieu Salzmann
Co-examiner: Prof. Pierre Dillenbourg
Abstract
Structured representations, such as Bags of Words, VLAD and Fisher Vectors, have proven highly successful to tackle complex visual recognition tasks. As such, they have been incorporated into deep architectures. Our goal is to develop deep attentional architectures that jointly learn an interpretable semantic codebook and a structured representation of the input image to better understand of the way deep networks perform visual recognition tasks. Our framework is designed to understand how networks make predictions, as well as when and why they make errors. We leverage the semantic codebooks to detect malicious inputs in case of adversarial attacks and propose a defense system to tackle the same. We first show the effectiveness of structured representations for the task of large scale image retrieval. We then present a visualization technique using generator networks to interpret the hidden neurons of a deep network and finally discuss a state-of-the-art method on visual attention system that focus on discriminative regions of the image. We conclude with the current state of results and directions for future research.
Background papers
Aggregating local descriptors into compact codes, PAMI 2012
Synthesizing the preferred inputs for neurons in neural networks via deep generator networks, NIPS 2016
Attentional Pooling for Action Recognition, NIPS 2017.
Exam president: Prof. Sabine Süsstrunk
Thesis advisor: Prof. Pascal Fua
Thesis co-advisor: Dr Matthieu Salzmann
Co-examiner: Prof. Pierre Dillenbourg
Abstract
Structured representations, such as Bags of Words, VLAD and Fisher Vectors, have proven highly successful to tackle complex visual recognition tasks. As such, they have been incorporated into deep architectures. Our goal is to develop deep attentional architectures that jointly learn an interpretable semantic codebook and a structured representation of the input image to better understand of the way deep networks perform visual recognition tasks. Our framework is designed to understand how networks make predictions, as well as when and why they make errors. We leverage the semantic codebooks to detect malicious inputs in case of adversarial attacks and propose a defense system to tackle the same. We first show the effectiveness of structured representations for the task of large scale image retrieval. We then present a visualization technique using generator networks to interpret the hidden neurons of a deep network and finally discuss a state-of-the-art method on visual attention system that focus on discriminative regions of the image. We conclude with the current state of results and directions for future research.
Background papers
Aggregating local descriptors into compact codes, PAMI 2012
Synthesizing the preferred inputs for neurons in neural networks via deep generator networks, NIPS 2016
Attentional Pooling for Action Recognition, NIPS 2017.
Practical information
- General public
- Free
Contact
- EDIC - edic@epfl.ch