Dr. Aaron Mueller: Mechanistically Controlling Language Models
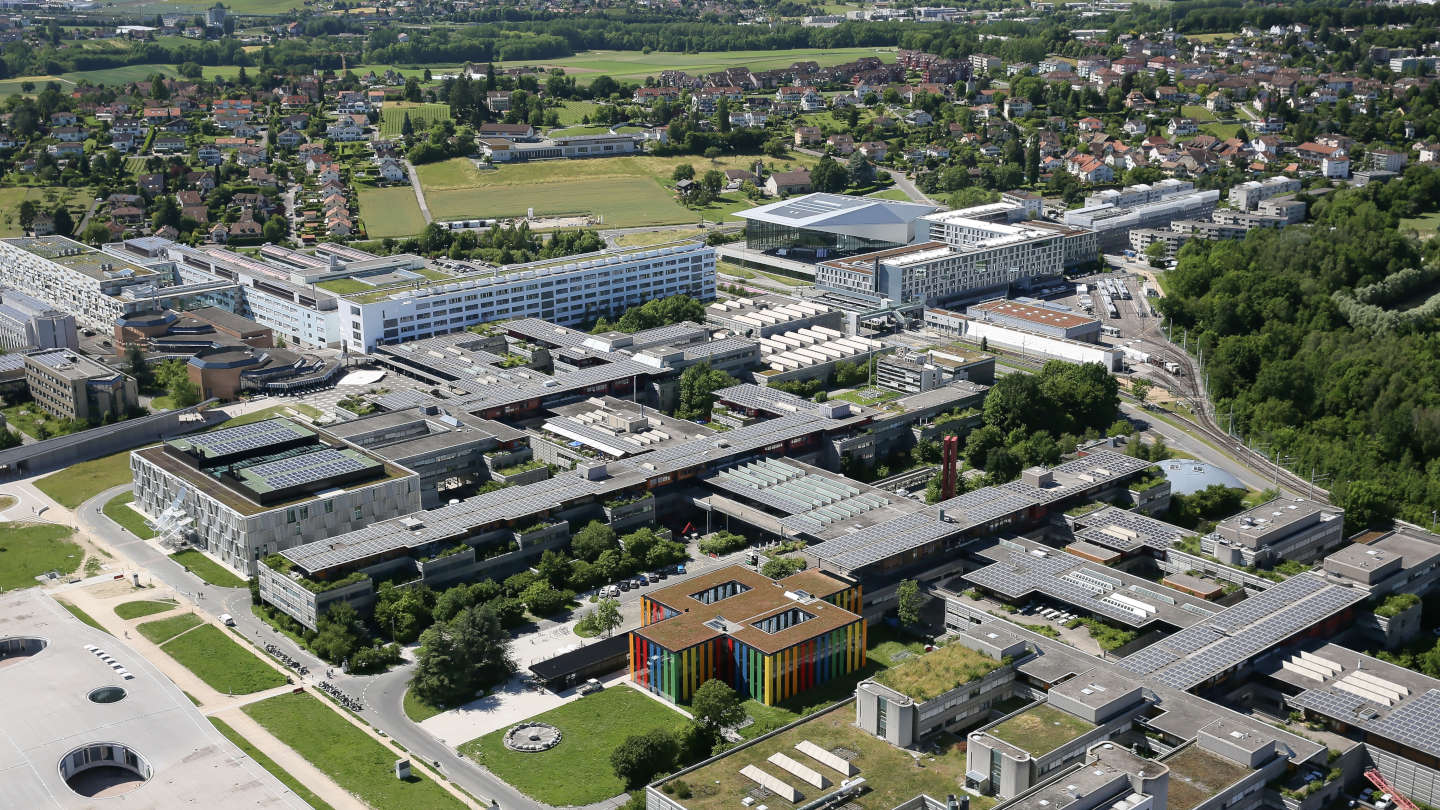
Event details
Date | 04.07.2024 |
Hour | 11:00 › 12:00 |
Speaker | Dr. Aaron Mueller |
Location | |
Category | Conferences - Seminars |
Event Language | English |
Abstract
Language models (LMs) often generalize in unpredictable ways. Mechanistic interpretability has recently received significant attention as a way to better understand how these surprisingly capable systems arrive at their behaviors. However, aside from scientific interest and understanding, what are the practical implications of interpretability findings? Can we use the results of interpretability studies to directly control how language models generalize? In this talk, I will describe two recent efforts toward understanding and precisely controlling model behaviors. I will start by describing function vectors; these are linear representations of input-output functions derived from the hidden states of language models. I will discuss two interesting properties of function vectors: (1) they can be composed to trigger more complex task execution in a zero-shot manner, and (2) they generalize well outside the distribution on which they were discovered. Then, I will describe sparse feature circuits; these are causally implicated subnetworks of human-interpretable features. I will demonstrate an application of sparse feature circuits where we ablate irrelevant features from a human-interpretable circuit to surgically improve the generalization of a classifier. I will conclude by discussing opportunities and challenges in using mechanistic insights to control language models.
Bio
Aaron Mueller is a Zuckerman postdoctoral fellow at Northeastern University, and an incoming assistant professor at Boston University in 2025. His work spans topics in the intersection of natural language processing, interpretability, and psycholinguistics, including causal and mechanistic interpretability methods, sample-efficient pretraining, and evaluations inspired by linguistic principles. He obtained his PhD from Johns Hopkins University in 2023, supervised by Tal Linzen. He was an NSF Graduate Fellow, and has received an Outstanding Paper Award from ACL (2023), a Featured Paper recognition from TMLR (2023), and coverage in the New York Times as an organizer of the BabyLM Challenge.
Practical information
- Informed public
- Free
Organizer
- Professor Antoine Bosselut
Contact
- Gail Weiss ([email protected])