External FLAIR seminar: Spencer Frei
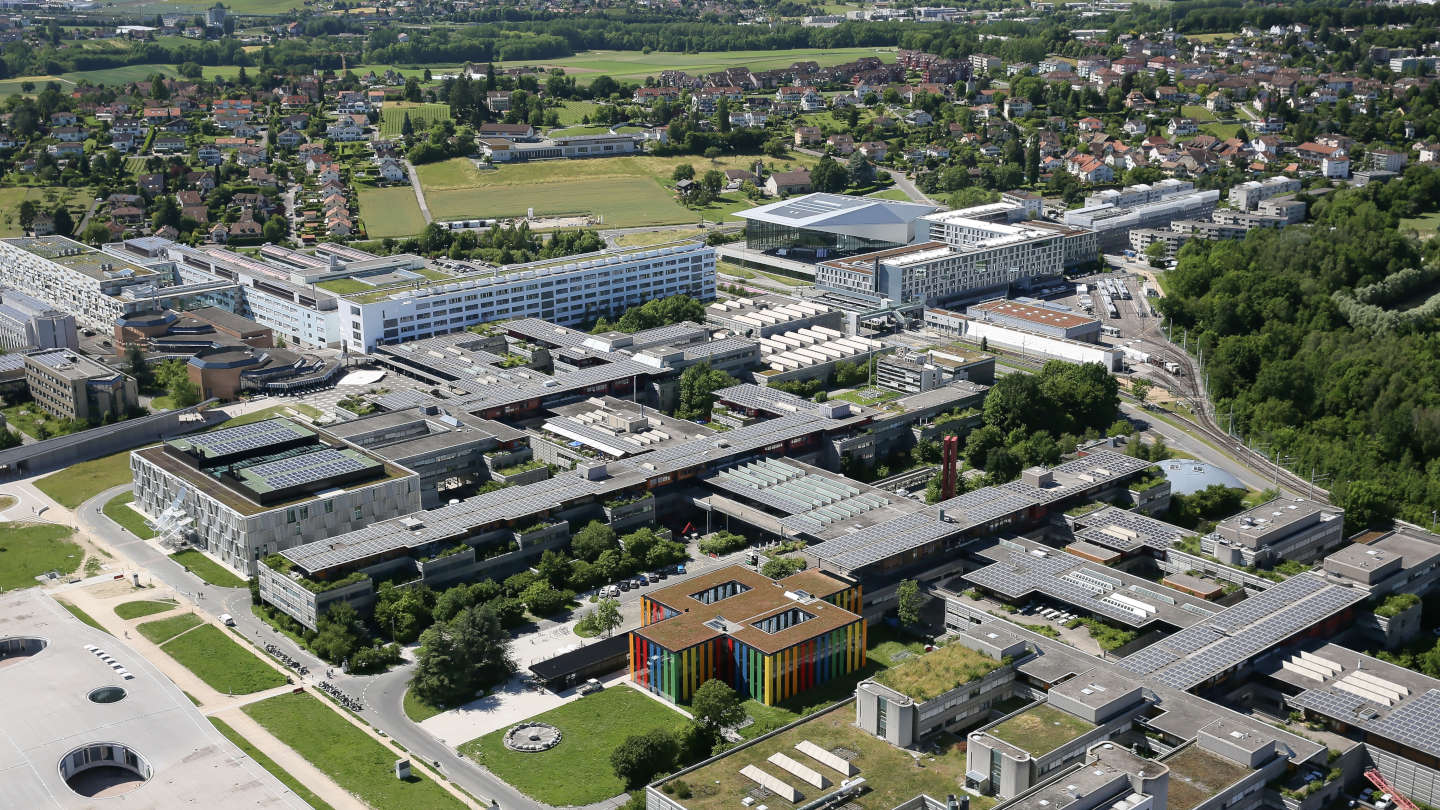
Event details
Date | 30.09.2022 |
Hour | 13:15 › 14:15 |
Speaker | Spencer Frei |
Location | |
Category | Conferences - Seminars |
Event Language | English |
Title: Implicit bias and benign overfitting for neural networks in high dimensions
Speaker: Spencer Frei (UC Berkeley)
Abstract: Benign overfitting, the phenomenon where interpolating models generalize well in the presence of noisy data, was first observed in neural networks trained by gradient descent. In this talk we go over some recent work towards understanding this surprising phenomenon. We first describe an implicit regularization effect of gradient descent in two-layer neural networks when trained on high-dimensional datasets. We show that in this setting, gradient descent finds solutions which have small rank, despite the lack of explicit regularization to encourage such structure. We then consider the generalization error of trained two-layer networks when the data comes from a high-dimensional mixture model where a constant fraction of the training labels are uniformly random labels. In this setting, we show that neural networks indeed exhibit benign overfitting: they can be driven to zero training error, perfectly fitting the noisy training labels, and simultaneously achieve minimax-optimal test error. In contrast to previous work on benign overfitting that require linear or kernel-based predictors, our analysis holds in a setting where both the model and learning dynamics are fundamentally nonlinear. Based on previous and upcoming work with Peter Bartlett, Niladri Chatterji, Wei Hu, Nati Srebro, and Gal Vardi.
Speaker: Spencer Frei (UC Berkeley)
Abstract: Benign overfitting, the phenomenon where interpolating models generalize well in the presence of noisy data, was first observed in neural networks trained by gradient descent. In this talk we go over some recent work towards understanding this surprising phenomenon. We first describe an implicit regularization effect of gradient descent in two-layer neural networks when trained on high-dimensional datasets. We show that in this setting, gradient descent finds solutions which have small rank, despite the lack of explicit regularization to encourage such structure. We then consider the generalization error of trained two-layer networks when the data comes from a high-dimensional mixture model where a constant fraction of the training labels are uniformly random labels. In this setting, we show that neural networks indeed exhibit benign overfitting: they can be driven to zero training error, perfectly fitting the noisy training labels, and simultaneously achieve minimax-optimal test error. In contrast to previous work on benign overfitting that require linear or kernel-based predictors, our analysis holds in a setting where both the model and learning dynamics are fundamentally nonlinear. Based on previous and upcoming work with Peter Bartlett, Niladri Chatterji, Wei Hu, Nati Srebro, and Gal Vardi.
Practical information
- Informed public
- Free
Contact
- Lénaïc Chizat: lenaic.chizat@epfl.ch François Ged: francois.ged@epfl.ch