External FLAIR seminar : Theodor Misiakiewicz
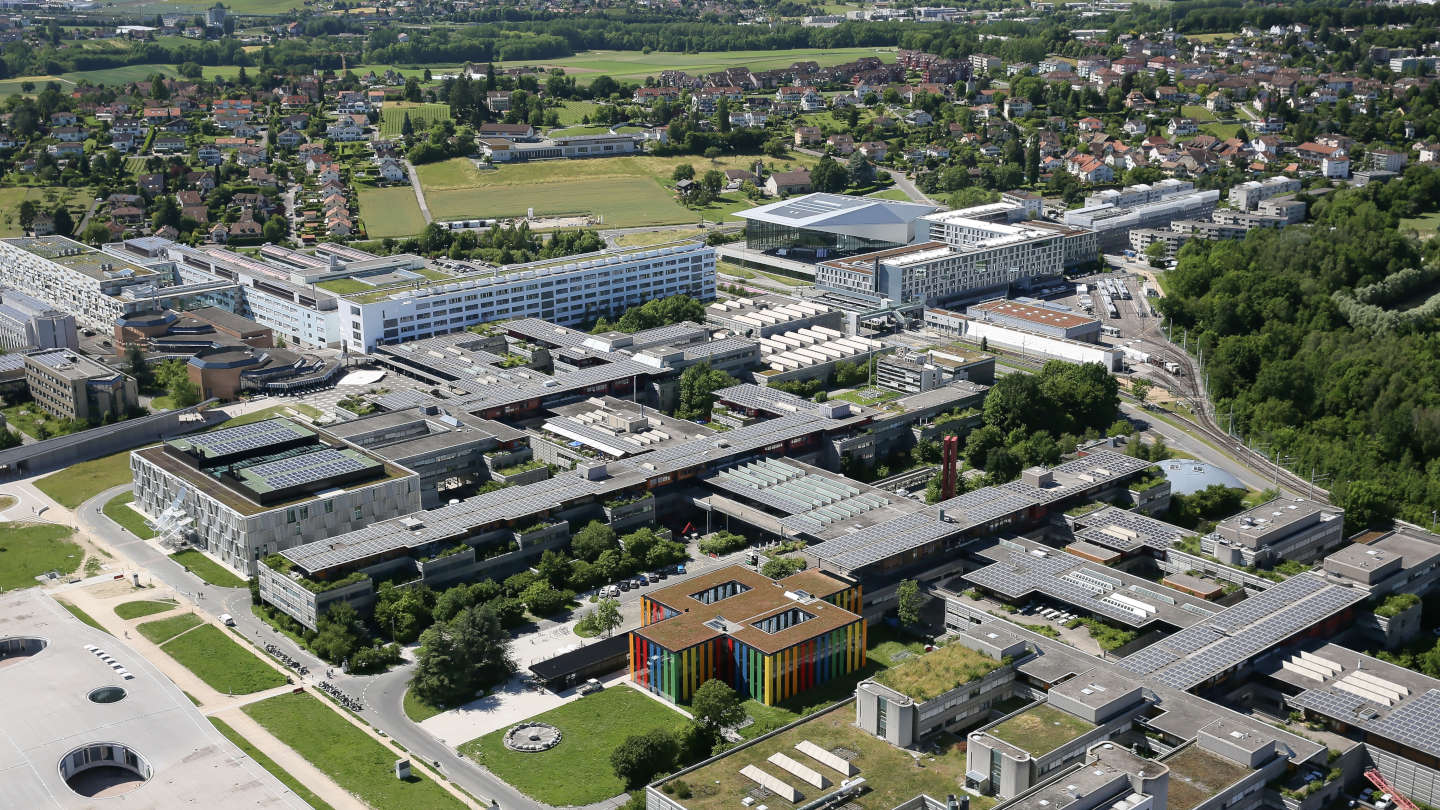
Event details
Date | 16.09.2022 |
Hour | 13:15 › 14:15 |
Speaker | Theodor Misiakiewicz |
Location | |
Category | Conferences - Seminars |
Event Language | English |
Title: Learning sparse functions with neural networks
Speaker: Theodor Misiakiewicz (Stanford University)
Abstract: Understanding deep learning requires to understand three components: approximation (number of parameters to approximate a target function), generalization (number of samples to generalize to unseen data) and computation (typically gradient-based optimization, number of iterations). However, studying their interplay remains a formidable challenge and led to the introduction of many new ideas (implicit regularization, tractability via overparametrization, benign overfitting etc.).
This talk will focus on the setting of learning sparse functions (a function that depends on a latent low-dimensional subspace) on the hypersphere or hypercube. I will consider three scenarios corresponding to three optimization regimes of neural networks (NNs): 1) kernel and random feature regression; 2) convex NNs; and 3) online SGD on 2-layer NNs in the mean-field scaling. In each of these scenarios, we provide tight characterizations for each of the approximation, generalization and computational aspects. In particular, while NNs trained beyond the kernel regime can adapt to sparsity, computational aspects cannot be ignored. Understanding which sparse functions are efficiently learned by NNs reveals interesting hierarchical structures in the target function (staircase property) and rich behavior in the SGD dynamics (saddles).
This is based on a few joint works with Emmanuel Abbe, Enric Boix-Adsera, Michael Celentano, Behrooz Ghorbani, Hong Hu, Yue M. Lu, Song Mei and Andrea Montanari.
Practical information
- Informed public
- Free
Contact
- Lénaïc Chizat: [email protected] François Ged: [email protected]