Harmonic analysis of deep convolutional neural networks
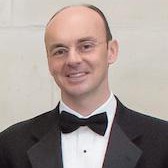
Event details
Date | 27.02.2017 |
Hour | 14:00 › 15:00 |
Speaker | Prof. Helmut Bölcskei - ETH Zürich - Communication Technology Laboratory |
Location | |
Category | Conferences - Seminars |
Deep convolutional neural networks (DCNNs) have led to breakthrough results in numerous machine learning tasks, yet a comprehensive mathematical theory explaining this success seems distant.
In this talk, we explain how the structure of DCNNs naturally leads to desirable invariance and deformation insensitivity properties for feature extraction and classification.
We then establish formally that deep neural networks with arbitrary convolution kernels, general Lipschitz-continuous non-linearities (e.g., rectified linear units, shifted logistic sigmoids, hyperbolic tangents, and modulus functions), and a wide range of pooling operators exhibit vertical translation invariance and small deformation sensitivity. We also analyze energy conservation and energy decay properties of DCNNs and identify networks with exponential energy decay. In particular, we provide estimates on the number of layers needed to have most of the input signal energy be contained in the network’s feature maps. On a conceptual level our results establish that deformation insensitivity, vertical translation invariance, and energy conservation are guaranteed by the network structure per se rather than the specific convolution kernels, non-linearities, and pooling operators. This offers a mathematical explanation for certain aspects of the tremendous practical success of DCNNs.
Practical information
- Informed public
- Free
- This event is internal
Organizer
- Prof. Michael Unser - Biomedical Imaging Group