Hierarchical Community Detection
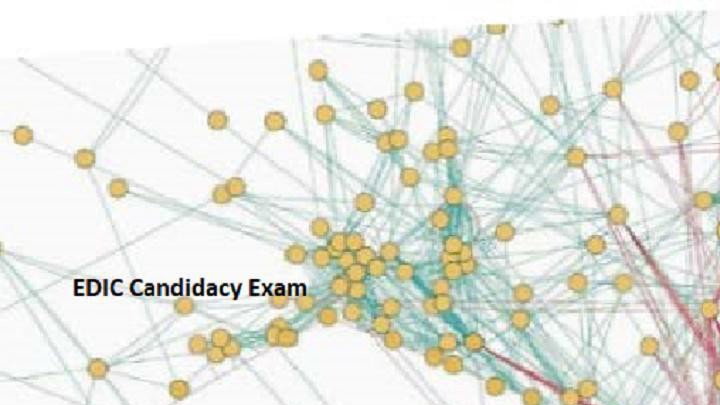
Event details
Date | 01.07.2024 |
Hour | 13:00 › 15:00 |
Speaker | Daichi Kuroda |
Location | |
Category | Conferences - Seminars |
EDIC candidacy exam
Exam president: Prof. Pascal Frossard
Thesis advisor: Prof. Patrick Thiran
Thesis co-advisor: Prof. Matthias Grossglauser
Co-examiner: Prof. Sofia Olhede
Abstract
Hierarchical community detection is a task to identify the communities within a network and their hierarchical organization, typically resulting in a rooted tree output.
We first examine a top-down method that achieves a near information-theoretical threshold for recovering communities in a particular case of the hierarchical stochastic block model (HSBM)-a class of random graphs with planted hierarchical communities. Then, we discuss the most popular objective function for assessing algorithm performance beyond HSBMs.
Finally, we address current research's limitations, specifically the absence of a clear definition of hierarchy and methods to handle non-binary hierarchies and provide an outlook on addressing these limitations.
Background papers
1) Li, T., Lei, L., Bhattacharyya, S., Van den Berge, K., Sarkar, P., Bickel, P.J. and Levina, E., 2022. Hierarchical community detection by recursive partitioning. Journal of the American Statistical Association, 117(538), pp.951-968. https://doi.org/10.1080/01621459.2020.1833888
2) Dasgupta, S., 2016. A cost function for similarity-based hierarchical clustering. In Proceedings of the forty-eighth annual ACM symposium on Theory of Computing (pp. 118-127). https://doi.org/10.1145/2897518.2897527
3) Cohen-Addad, V., Kanade, V. and Mallmann-Trenn, F., 2017. Hierarchical clustering beyond the worst-case. Advances in Neural Information Processing Systems, 30. https://proceedings.neurips.cc/paper_files/paper/2017/file/e8bf0f27d70d480d3ab793bb7619aaa5-Paper.pdf
Exam president: Prof. Pascal Frossard
Thesis advisor: Prof. Patrick Thiran
Thesis co-advisor: Prof. Matthias Grossglauser
Co-examiner: Prof. Sofia Olhede
Abstract
Hierarchical community detection is a task to identify the communities within a network and their hierarchical organization, typically resulting in a rooted tree output.
We first examine a top-down method that achieves a near information-theoretical threshold for recovering communities in a particular case of the hierarchical stochastic block model (HSBM)-a class of random graphs with planted hierarchical communities. Then, we discuss the most popular objective function for assessing algorithm performance beyond HSBMs.
Finally, we address current research's limitations, specifically the absence of a clear definition of hierarchy and methods to handle non-binary hierarchies and provide an outlook on addressing these limitations.
Background papers
1) Li, T., Lei, L., Bhattacharyya, S., Van den Berge, K., Sarkar, P., Bickel, P.J. and Levina, E., 2022. Hierarchical community detection by recursive partitioning. Journal of the American Statistical Association, 117(538), pp.951-968. https://doi.org/10.1080/01621459.2020.1833888
2) Dasgupta, S., 2016. A cost function for similarity-based hierarchical clustering. In Proceedings of the forty-eighth annual ACM symposium on Theory of Computing (pp. 118-127). https://doi.org/10.1145/2897518.2897527
3) Cohen-Addad, V., Kanade, V. and Mallmann-Trenn, F., 2017. Hierarchical clustering beyond the worst-case. Advances in Neural Information Processing Systems, 30. https://proceedings.neurips.cc/paper_files/paper/2017/file/e8bf0f27d70d480d3ab793bb7619aaa5-Paper.pdf
Practical information
- General public
- Free