Information-theoretic perspectives on active sampling
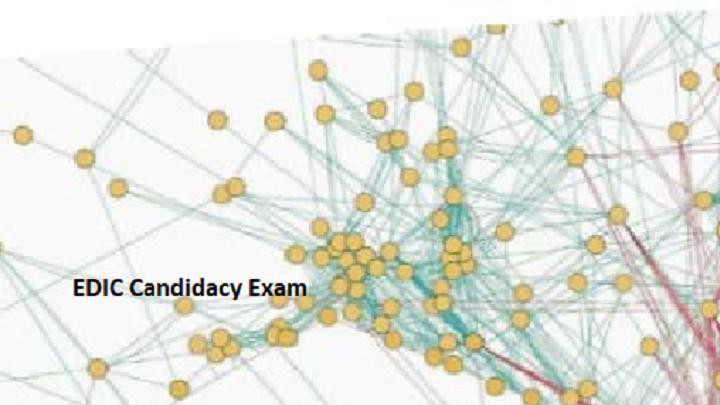
Event details
Date | 25.06.2024 |
Hour | 13:00 › 15:00 |
Speaker | Millen Kanabar |
Location | |
Category | Conferences - Seminars |
EDIC candidacy exam
Exam president: Prof. Rüdiger Urbanke
Thesis advisor: Prof. Michael Gastpar
Co-examiner: Prof. Nicolas Flammarion
Abstract
In this proposal, we consider agents that can decide upon actions sequentially in three different pure exploration settings: an agent that wishes to find via sampling, with fixed confidence, the distribution with the highest mean from a subset of an exponential family; an agent that wishes to infer the correct hypothesis under limited information; and finally, an agent that wishes to best replicate an optimal binary classifier with an option to abstain for a fixed penalty. We compare the sampling models, the strategies discussed along with matching lower bounds in each case and comment on future directions of work.
Background papers
Exam president: Prof. Rüdiger Urbanke
Thesis advisor: Prof. Michael Gastpar
Co-examiner: Prof. Nicolas Flammarion
Abstract
In this proposal, we consider agents that can decide upon actions sequentially in three different pure exploration settings: an agent that wishes to find via sampling, with fixed confidence, the distribution with the highest mean from a subset of an exponential family; an agent that wishes to infer the correct hypothesis under limited information; and finally, an agent that wishes to best replicate an optimal binary classifier with an option to abstain for a fixed penalty. We compare the sampling models, the strategies discussed along with matching lower bounds in each case and comment on future directions of work.
Background papers
- Cecchi, Fabio, and Nidhi Hegde. 2017. “Adaptive Active Hypothesis Testing under Limited Information.” In Advances in Neural Information Processing Systems. Vol. 30. Curran Associates, Inc.https://proceedings.neurips.cc/paper_files/paper/2017/hash/9f44e956e3a2b7b5598c625fcc802c36-Abstract.html
- Garivier, Aurélien, and Emilie Kaufmann. 2016. “Optimal Best Arm Identification with Fixed Confidence.” In Conference on Learning Theory, 998–1027. PMLR.https://proceedings.mlr.press/v49/garivier16a.htm
- Shekhar, Shubhanshu, Mohammad Ghavamzadeh, and Tara Javidi. 2020. “Active Learning for Classification with Abstention.” In 2020 IEEE International Symposium on Information Theory (ISIT), 2801–6. https://doi.org/10.1109/ISIT44484.2020.9174242.
Practical information
- General public
- Free