Insights on the generalization ability of deep neural networks using sensitivity analysis
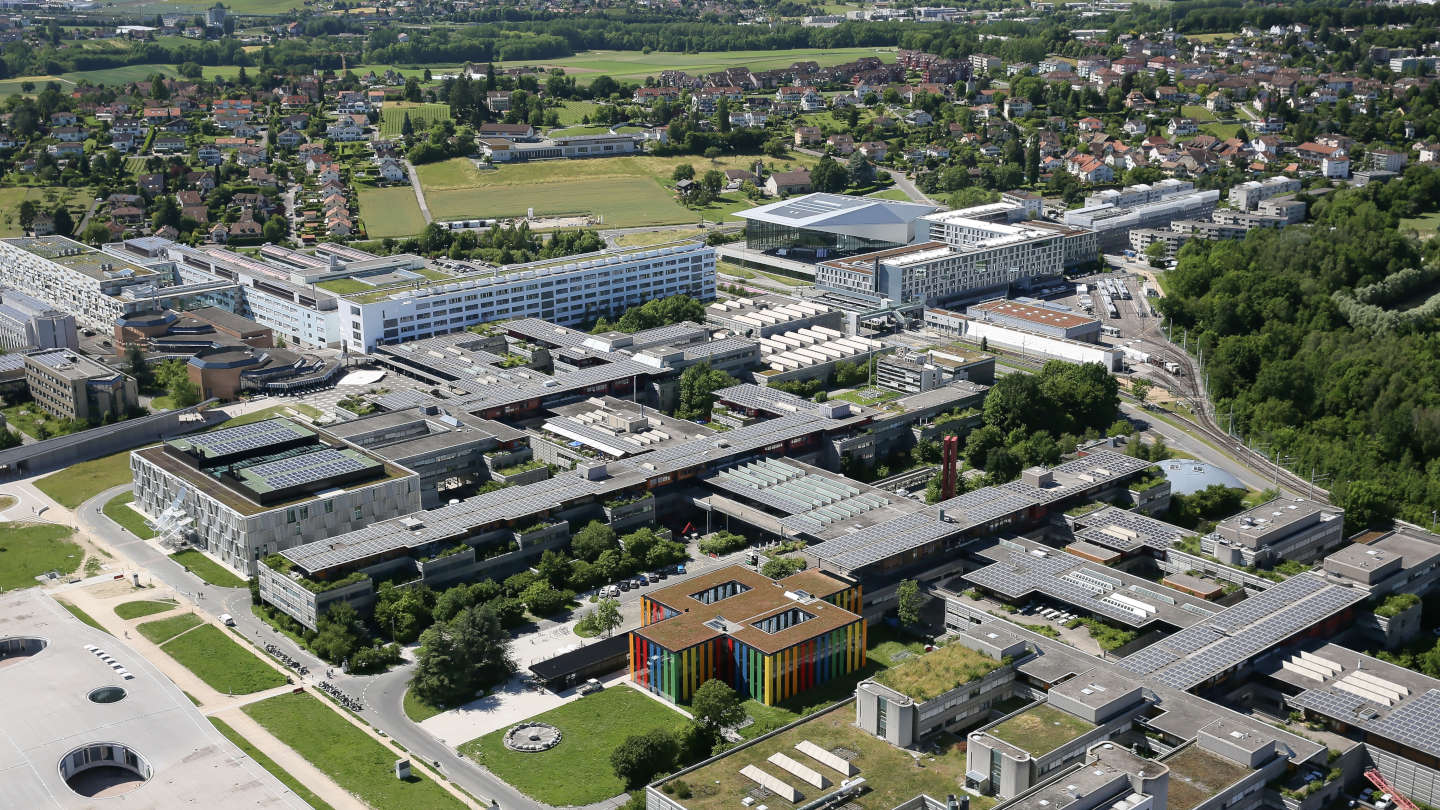
Event details
Date | 28.06.2018 |
Hour | 10:00 › 12:00 |
Speaker | Mahsa Forouzesh |
Location | |
Category | Conferences - Seminars |
EDIC candidacy exam
Exam president: Prof. Ruediger Urbanke
Thesis advisor: Prof. Patrick Thiran
Co-examiner: Prof. Martin Jaggi
Abstract
There is a growing line of research on understanding what drives generalization in deep learning settings. Sharpness analysis of the loss surface gives intuition on the generalization process. Robustness analysis provides generalization error bounds which complexity measures of the model appear in. However, none is sufficient to explain the generalization ability of an over-parameterized deep neural network to unseen data. In this proposal, we would like to find insights on tackling this phenomenon using mathematical tools. In particular, we would like to apply sensitivity analysis to both forward pass and backward pass of the model, and by considering a probabilistic framework, we would like to provide a better understanding of the performance of various algorithms. Theoretical explanation on why and how deep neural networks work is the starting point for designing new regularization techniques that are not only justified by empirical results but also have mathematical fundamentals.
Background papers
Mathematics of Deep Learning, by Vidal, R., et al.
Entropy-SGD: Biasing gradient descent into wide valleys, by Chaudhari, P., et al.
Layer Normalization, by Lei Ba, J., et al.
Exam president: Prof. Ruediger Urbanke
Thesis advisor: Prof. Patrick Thiran
Co-examiner: Prof. Martin Jaggi
Abstract
There is a growing line of research on understanding what drives generalization in deep learning settings. Sharpness analysis of the loss surface gives intuition on the generalization process. Robustness analysis provides generalization error bounds which complexity measures of the model appear in. However, none is sufficient to explain the generalization ability of an over-parameterized deep neural network to unseen data. In this proposal, we would like to find insights on tackling this phenomenon using mathematical tools. In particular, we would like to apply sensitivity analysis to both forward pass and backward pass of the model, and by considering a probabilistic framework, we would like to provide a better understanding of the performance of various algorithms. Theoretical explanation on why and how deep neural networks work is the starting point for designing new regularization techniques that are not only justified by empirical results but also have mathematical fundamentals.
Background papers
Mathematics of Deep Learning, by Vidal, R., et al.
Entropy-SGD: Biasing gradient descent into wide valleys, by Chaudhari, P., et al.
Layer Normalization, by Lei Ba, J., et al.
Practical information
- General public
- Free
Contact
- EDIC - edic@epfl.ch