Learning Light Scattering Distributions
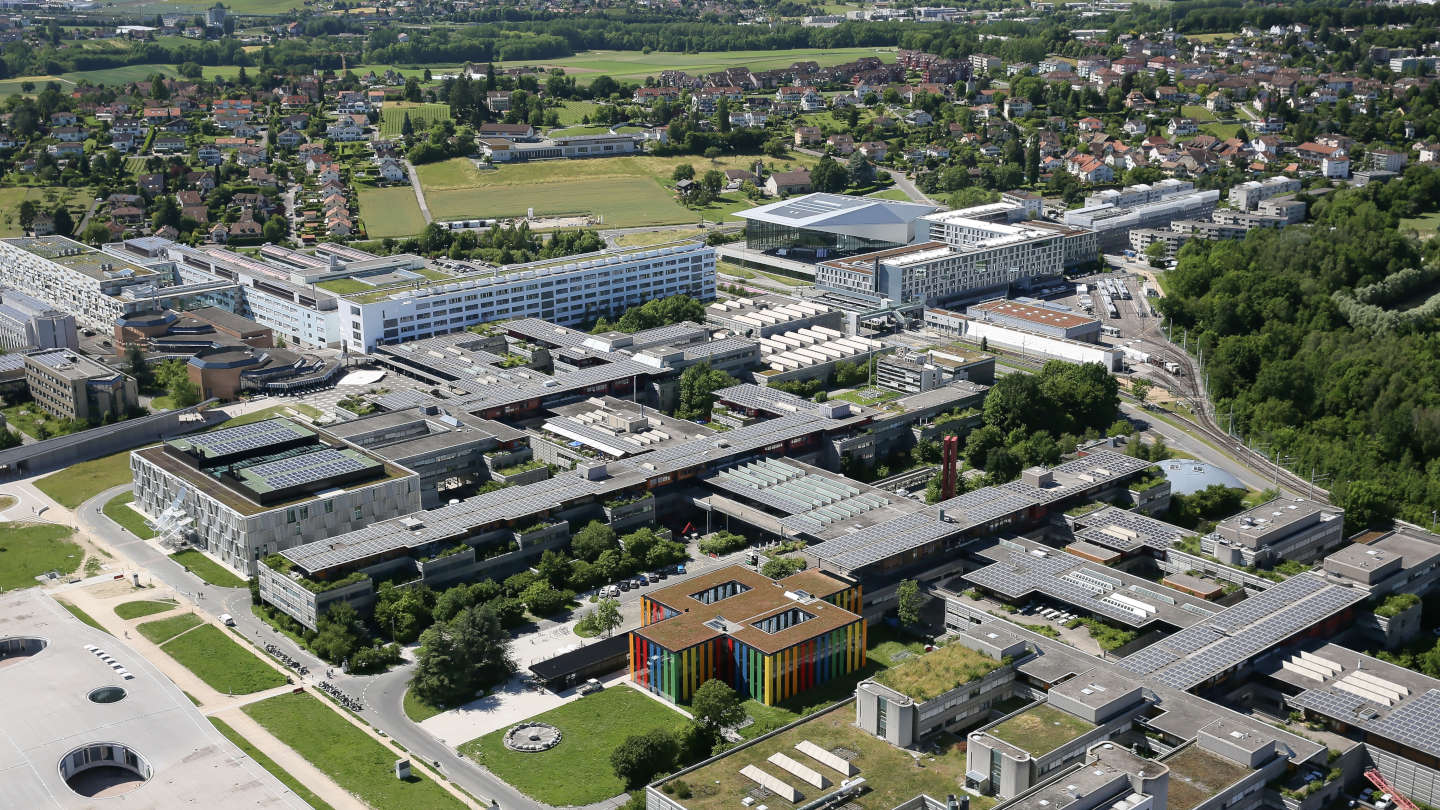
Event details
Date | 30.08.2018 |
Hour | 09:30 › 11:30 |
Speaker | Delio Vicini |
Location | |
Category | Conferences - Seminars |
EDIC candidacy exam
Exam president: Prof. Pascal Fua
Thesis advisor: Prof. Wenzel Jakob
Co-examiner: Prof. Mark Pauly
Abstract
Rendering photorealistic imagery remains challenging, as ever growing
demands on visual fidelity quickly make up for any gains in hardware performance
and algorithmic improvements. Our research objective is to further
reduce the time required to render complex light transport effects by using
machine learning to model the distribution of scattered light. In this proposal,
we summarize three related previous papers. First, we describe a paper on
the efficient rendering of subsurface scattering. We then summarize a paper
introducing variational autoencoders, a generative neural network architecture,
which allows to learn how to sample from arbitrary distributions. The
third paper presents an online learning method to learn the radiance distribution
during rendering. We further present a novel approach to rendering
subsurface scattering using a variational autoencoder.
In the future, we could apply a similar architecture to more general light transport problems.
Background papers
Photon Beam Diffusion: A Hybrid Monte Carlo Method for Subsurface Scattering, by Habel et al.
Auto-Encoding Variational Bayes, by Kingma and Welling
Practical Path Guiding for Efficient Light-Transport Simulation, by Müller et al.
Exam president: Prof. Pascal Fua
Thesis advisor: Prof. Wenzel Jakob
Co-examiner: Prof. Mark Pauly
Abstract
Rendering photorealistic imagery remains challenging, as ever growing
demands on visual fidelity quickly make up for any gains in hardware performance
and algorithmic improvements. Our research objective is to further
reduce the time required to render complex light transport effects by using
machine learning to model the distribution of scattered light. In this proposal,
we summarize three related previous papers. First, we describe a paper on
the efficient rendering of subsurface scattering. We then summarize a paper
introducing variational autoencoders, a generative neural network architecture,
which allows to learn how to sample from arbitrary distributions. The
third paper presents an online learning method to learn the radiance distribution
during rendering. We further present a novel approach to rendering
subsurface scattering using a variational autoencoder.
In the future, we could apply a similar architecture to more general light transport problems.
Background papers
Photon Beam Diffusion: A Hybrid Monte Carlo Method for Subsurface Scattering, by Habel et al.
Auto-Encoding Variational Bayes, by Kingma and Welling
Practical Path Guiding for Efficient Light-Transport Simulation, by Müller et al.
Practical information
- General public
- Free
Contact
- EDIC - edic@epfl.ch