Pluralistic Foundation Model Alignment
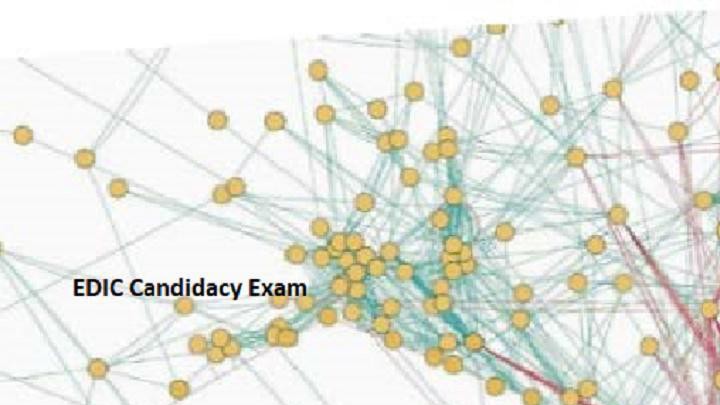
Event details
Date | 28.06.2024 |
Hour | 13:00 › 15:00 |
Speaker | Mikhail Terekhov |
Location | |
Category | Conferences - Seminars |
Event Language | English |
EDIC candidacy exam
Exam president: Prof. Robert West
Thesis advisor: Prof. Caglar Gulcehre
Co-examiner: Prof. Martin Jaggi
Abstract
Foundation models are machine learning models that were trained on a large and diverse dataset so that they can be quickly fine-tuned or used directly for many tasks. We consider the problem of pluralistic alignment of foundation models, with the goal of making the models more inclusive and giving the users more fine-grained control over the outputs. Given existing techniques for alignment, this problem is naturally formulated in the context of Multi-Objective Reinforcement Learning (MORL). Our research will therefore focus on methods for MORL and their application to alignment of foundation models. We will investigate extensions of popular on-policy algorithms for reinforcement learning, such as A2C and PPO, to the multi-objective case. Our methods will cover both discrete and continuous action spaces. The developed methods for MORL will be applied to perform multi-objective alignment using a generalization of RLHF. Concurrently, RL-free approaches to multi-objective alignment will also be considered. We will collaborate with social sciences to design methods for collecting data incorporating diverse perspectives from different sociocultural groups and to design ways of evaluating the plurality of our models.
Background papers
1. Ouyang, Long, et al. "Training language models to follow instructions with human feedback." Advances in Neural Information Processing Systems 35 (2022): 27730-27744. https://arxiv.org/abs/2203.0215
2. Xu, Jie, et al. "Prediction-guided multi-objective reinforcement learning for continuous robot control." International conference on machine learning. PMLR, 2020. https://proceedings.mlr.press/v119/xu20h.html
3. Rafailov, Rafael, et al. "Direct preference optimization: Your language model is secretly a reward model." Advances in Neural Information Processing Systems 36 (2024). https://arxiv.org/abs/2305.18290
Exam president: Prof. Robert West
Thesis advisor: Prof. Caglar Gulcehre
Co-examiner: Prof. Martin Jaggi
Abstract
Foundation models are machine learning models that were trained on a large and diverse dataset so that they can be quickly fine-tuned or used directly for many tasks. We consider the problem of pluralistic alignment of foundation models, with the goal of making the models more inclusive and giving the users more fine-grained control over the outputs. Given existing techniques for alignment, this problem is naturally formulated in the context of Multi-Objective Reinforcement Learning (MORL). Our research will therefore focus on methods for MORL and their application to alignment of foundation models. We will investigate extensions of popular on-policy algorithms for reinforcement learning, such as A2C and PPO, to the multi-objective case. Our methods will cover both discrete and continuous action spaces. The developed methods for MORL will be applied to perform multi-objective alignment using a generalization of RLHF. Concurrently, RL-free approaches to multi-objective alignment will also be considered. We will collaborate with social sciences to design methods for collecting data incorporating diverse perspectives from different sociocultural groups and to design ways of evaluating the plurality of our models.
Background papers
1. Ouyang, Long, et al. "Training language models to follow instructions with human feedback." Advances in Neural Information Processing Systems 35 (2022): 27730-27744. https://arxiv.org/abs/2203.0215
2. Xu, Jie, et al. "Prediction-guided multi-objective reinforcement learning for continuous robot control." International conference on machine learning. PMLR, 2020. https://proceedings.mlr.press/v119/xu20h.html
3. Rafailov, Rafael, et al. "Direct preference optimization: Your language model is secretly a reward model." Advances in Neural Information Processing Systems 36 (2024). https://arxiv.org/abs/2305.18290
Practical information
- General public
- Free