Special ENAC Research Day & EESS talk on "Multivariate Time Series Models for Environmental Applications based on Latent Stochastic Processes"
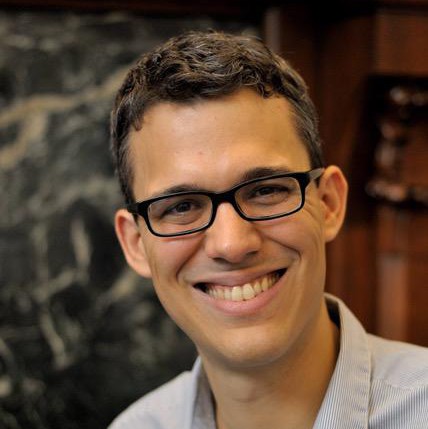
Event details
Date | 23.05.2017 |
Hour | 12:45 › 13:45 |
Speaker |
Dr Stéphane Guerrier, Assistant Professor, Department of Statistics, University of Illinois at Urbana-Champaign (USA) Short biography: Stéphane Guerrier received a M.Sc. degree in Environmental Engineering from the École Polytechnique Fédérale de Lausanne (EPFL), Switzerland, in 2008, and a Ph.D. degree in Statistics from the University of Geneva, Switzerland, in 2013. He is currently Assistant Professor with the Department of Statistics at the University of Illinois at Urbana–Champaign, IL, USA. His current research interests include time series analysis, spatial statistics, simulation-based estimation methods and robust statistics. |
Location | |
Category | Conferences - Seminars |
Abstract:
A growing area of interest in ecological research lies in the effects of changes in climate on biological communities and the effects of changes in biological communities on weather and climate. Climate conditions often are measured by ecologists as time series either with meteorological measurements recorded at weather stations or with environmental sensors at fixed time intervals. Mixed models are a good candidate to deal with these types of measurements allowing to obtain a better inference on the true parameters of interest for the researcher. However, the level of complexity which can be handled for the dependence structure in the residuals is limited in practice. Indeed, the examples in ecological research show how there can be a series of factors contributing to the dependence structure in residuals over time and the observations can be the result of different combinations of stochastic processes. Given these considerations, there is a need to model multivariate time series with a complex structure delivered by the fact that the individual time series can share some models and factors with some time series but not with others (or eventually not share any model at all). As a solution to this problem we present a method which addresses these issues based on the concept of latent processes which can be extended to a multivariate setting where the dependence between observations between and within factors can be explained by "shared'' models between time series. An approach based on this concept appears reasonable and indeed more appropriate considering the actual conditions under which experimental data is collected. In this work we use the idea of the Generalized Method of Wavelet Moments (GMWM) to estimate these multivariate time series with complex dependence structures. This is done in a straightforward and computationally efficient manner allowing to deliver an easy-to-implement method for the estimation of these random effects. When applied to some vapor pressure deficit data, the method provides results which are consistent with ecological interpretations and gives additional insight into the dependence structures among explanatory factors. This is a joint work with Allison Gardner, Roberto Molinari and James Balamuta.
A growing area of interest in ecological research lies in the effects of changes in climate on biological communities and the effects of changes in biological communities on weather and climate. Climate conditions often are measured by ecologists as time series either with meteorological measurements recorded at weather stations or with environmental sensors at fixed time intervals. Mixed models are a good candidate to deal with these types of measurements allowing to obtain a better inference on the true parameters of interest for the researcher. However, the level of complexity which can be handled for the dependence structure in the residuals is limited in practice. Indeed, the examples in ecological research show how there can be a series of factors contributing to the dependence structure in residuals over time and the observations can be the result of different combinations of stochastic processes. Given these considerations, there is a need to model multivariate time series with a complex structure delivered by the fact that the individual time series can share some models and factors with some time series but not with others (or eventually not share any model at all). As a solution to this problem we present a method which addresses these issues based on the concept of latent processes which can be extended to a multivariate setting where the dependence between observations between and within factors can be explained by "shared'' models between time series. An approach based on this concept appears reasonable and indeed more appropriate considering the actual conditions under which experimental data is collected. In this work we use the idea of the Generalized Method of Wavelet Moments (GMWM) to estimate these multivariate time series with complex dependence structures. This is done in a straightforward and computationally efficient manner allowing to deliver an easy-to-implement method for the estimation of these random effects. When applied to some vapor pressure deficit data, the method provides results which are consistent with ecological interpretations and gives additional insight into the dependence structures among explanatory factors. This is a joint work with Allison Gardner, Roberto Molinari and James Balamuta.
Practical information
- General public
- Free
- This event is internal
Organizer
- EESS - IIE
Contact
- Dr Jan Skaloud, TOPO