TBA
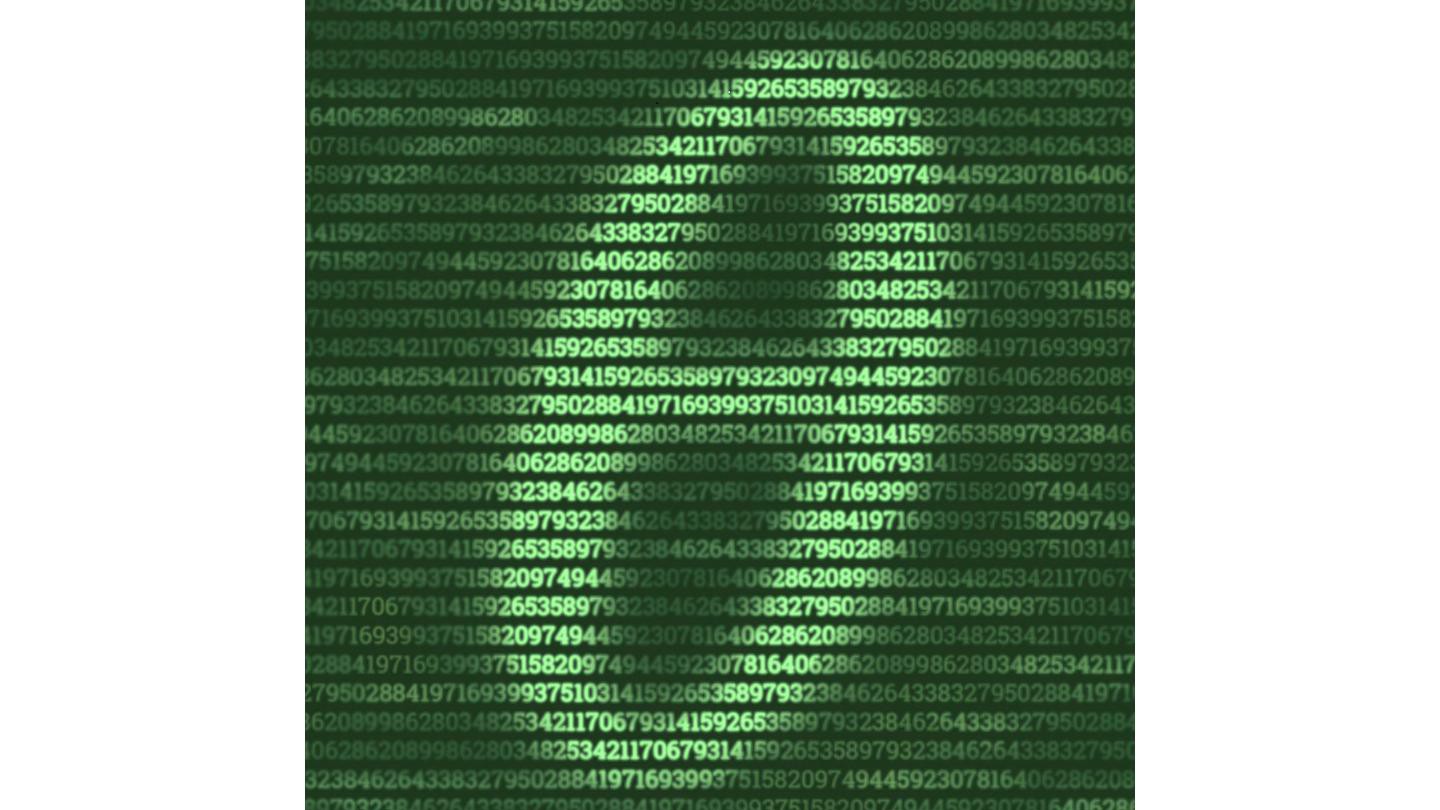
Event details
Date | 07.06.2024 |
Hour | 15:15 › 16:15 |
Speaker | Rainer von Sachs, UC Louvain |
Location | |
Category | Conferences - Seminars |
Event Language | English |
TBA
Practical information
- Informed public
- Free
Organizer
- Victor Panaretos
Contact
- Maroussia Schaffner