Weight Interpolation Techniques for Model Editing
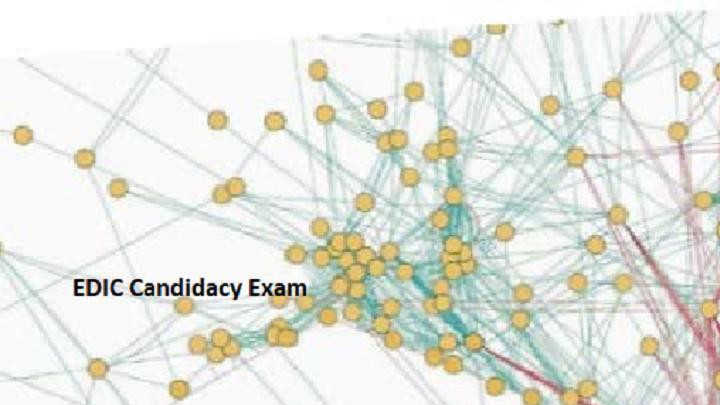
Event details
Date | 04.07.2024 |
Hour | 15:00 › 17:00 |
Speaker | Adam Hazimeh |
Location | |
Category | Conferences - Seminars |
EDIC candidacy exam
Exam president: Prof. Maria Brbic
Thesis advisor: Prof. Pascal Frossard
Co-examiner: Prof. Caglar Gulcehre
Abstract
Weight interpolation techniques have recently shown remarkable success in producing high-performing merged models. In this report, we first examine prior work that attributes their effectiveness to certain pre-training dynamics. We then explore model souping, a popular weight interpolation method, and task arithmetic, its multi-task extension. However, given that task arithmetic has only been studied in open-vocabulary models like CLIP, we extend its application to the closed-vocabulary setting. We experimentally investigate the effectiveness of closed-vocabulary task arithmetic and show that weight disentanglement - the property enabling task arithmetic - is a general consequence of neural network pre-training.
Background papers
Exam president: Prof. Maria Brbic
Thesis advisor: Prof. Pascal Frossard
Co-examiner: Prof. Caglar Gulcehre
Abstract
Weight interpolation techniques have recently shown remarkable success in producing high-performing merged models. In this report, we first examine prior work that attributes their effectiveness to certain pre-training dynamics. We then explore model souping, a popular weight interpolation method, and task arithmetic, its multi-task extension. However, given that task arithmetic has only been studied in open-vocabulary models like CLIP, we extend its application to the closed-vocabulary setting. We experimentally investigate the effectiveness of closed-vocabulary task arithmetic and show that weight disentanglement - the property enabling task arithmetic - is a general consequence of neural network pre-training.
Background papers
- Linear Mode Connectivity and the Lottery Ticket Hypothesis (https://arxiv.org/pdf/1912.05671.pdf)
- Model Soups: Averaging Weights of Multiple Fine-tuned Models Improves Accuracy without Increasing Inference Time (https://arxiv.org/pdf/2203.05482.pdf)
- Editing Models with Task Arithmetic (https://arxiv.org/pdf/2212.04089.pdf)
Practical information
- General public
- Free