CECAM workshop: "From machine-learning theory to driven complex systems and back"
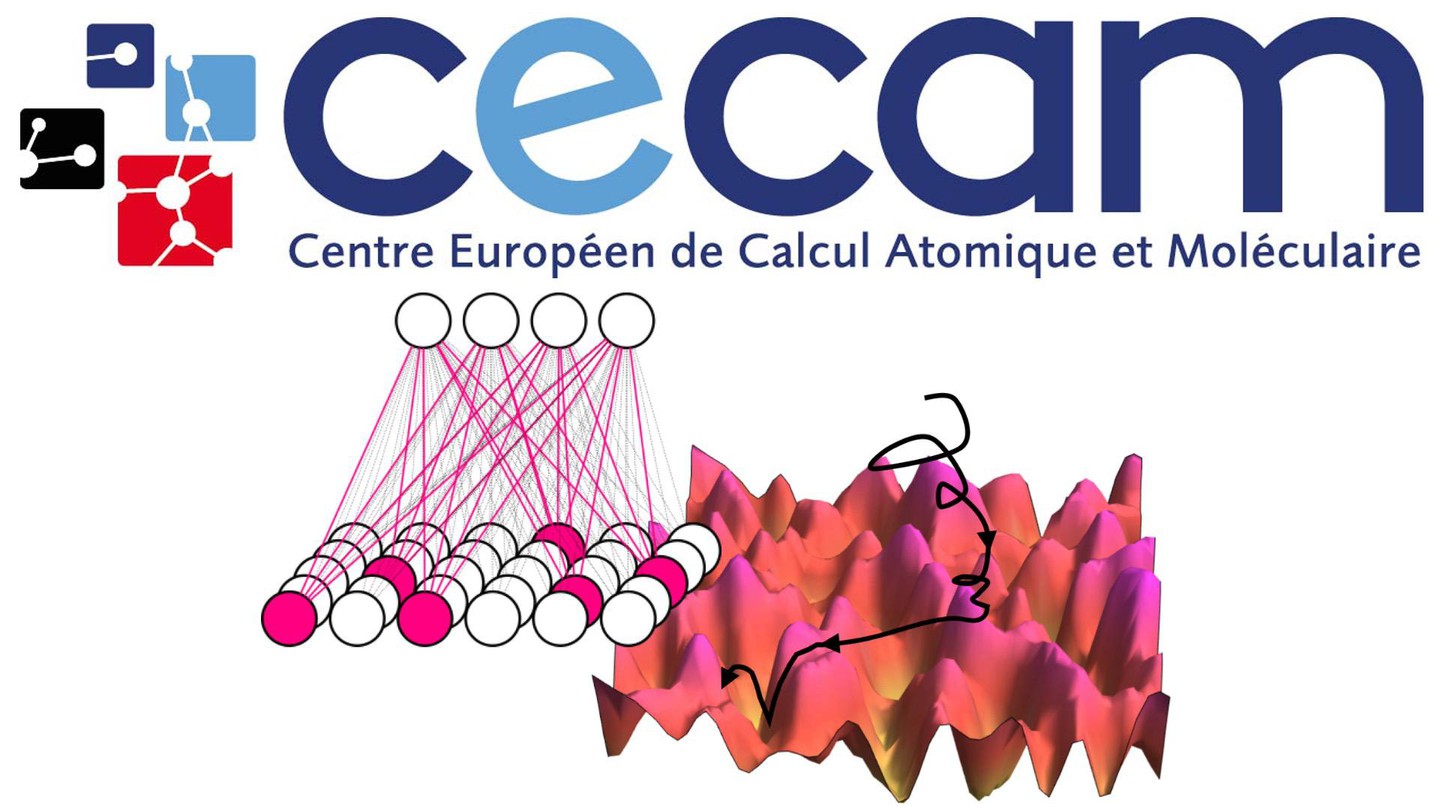
Event details
Date | 22.05.2024 › 24.05.2024 |
Location | |
Category | Conferences - Seminars |
Event Language | English |
You can apply to participate and find all the relevant information (speakers, abstracts, program,...) on the event website: https://www.cecam.org/workshop-details/1287
Description
In this workshop, we propose to gather researchers with complementary backgrounds, all involved in cutting-edge research in statistical physics, machine learning and statistical inference. The goal of this workshop is to strengthen the links between machine learning (ML), disordered systems and driven complex systems - such as structural glasses and dense active matter - to mutually exploit their theoretical and computational tools as well as their physical intuition. Our main focus will be on stochastic dynamical processes, out-of-equilibrium regimes and their insights into training dynamics, primarily from a computational perspective. In addition to deepening our theoretical understanding of the successes and limitations of ML, these connections will pave the way for the development of new algorithms and suggest alternative architectures.
We plan to address specifically the following topics:
- Dynamical Mean-Field Theory (DMFT)
- Generative neural networks for modeling
- Phase diagrams, landscapes and training optimization
Training a ML model with a particular architecture on a dataset amounts to evolving the parameters in a complex high-dimensional landscape defined by a given loss function. The main questions that arise are: (i) how the landscape statistical properties depend on the architecture and the dataset statistics, (ii) what is the associated performance of standard optimization algorithms such as stochastic gradient descent, (iii) how these algorithms can be improved in terms of generalization and computational efficiency, (iv) what is the impact of the dataset statistics on the learning process. In terms of modeling, a particular challenge is to design correlated artificial datasets to study the learning dynamics in a controlled manner. Moreover, important insights into either the learning process or practical applications should come from the interpretability of the learned parameters.
While it is obvious that deep neural networks are capable of handling increasingly complicated tasks, understanding how the formation of complex patterns relates to the dataset statistical properties is highly non-trivial, even for relatively simple architectures. Recent advances include the construction of novel loss functions aimed at accelerating the learning [4], the development of synthetic datasets with a higher degree of complexity capable of mimicking real datasets [5], or investigating the structure of the underlying complex landscapes [6]. In parallel, out-of-equilibrium physics has proven particularly useful in developing and controlling powerful generative models that can fully describe the variety of complex datasets [7,8,9,10]. These studies had a strong impact on the computational level. However, the development of new algorithms is often guided by intuitions about the loss-function specific properties, and a comprehensive understanding of the learning dynamics is still lacking.
Recent efforts in this direction rely on studying Langevin equations associated with simple models. The formalism of dynamical mean-field theory (DMFT), developed in the context of statistical physics to study the out-of-equilibrium dynamics of structural glasses [8,11] and even dense active systems [12], has been adapted to inference and ML models [13,14]. Its numerical implementation poses challenges that must be overcome to fully exploit it for improving the training process [15,16].
Finally, generative neural networks have great potential for modeling complex data. One approach is energy-based modeling, in which the probability distribution is represented by a Boltzmann distribution with a neural network as the energy function. Interpreting the trained neural network as a disordered interaction Hamiltonian is a powerful tool for inference applications [17,18]. However, further research is needed to understand how the dataset patterns are encoded in the model, and how to interpret them.
References
[1] Davide Carbone, Mengjian Hua, Simon Coste, Eric Vanden-Eijnden, "Efficient Training of Energy-Based Models Using Jarzynski Equality", arXiv:2305.19414 [cs.LG]
[2] A. Muntoni, A. Pagnani, M. Weigt, F. Zamponi, BMC Bioinformatics, 22, 528 (2021)
[3] S. Cocco, C. Feinauer, M. Figliuzzi, R. Monasson, M. Weigt, Rep. Prog. Phys., 81, 032601 (2018)
[4] Cedric Gerbelot, Emanuele Troiani, Francesca Mignacco, Florent Krzakala, Lenka Zdeborova, "Rigorous dynamical mean field theory for stochastic gradient descent methods", arXiv:2210.06591 [math-ph]
[5] A. Manacorda, G. Schehr, F. Zamponi, The Journal of Chemical Physics, 152, (2020)
[6] S. Sarao Mannelli, G. Biroli, C. Cammarota, F. Krzakala, P. Urbani, L. Zdeborová, Phys. Rev. X, 10, 011057 (2020)
[7] F. Mignacco, F. Krzakala, P. Urbani, L. Zdeborová, J. Stat. Mech., 2021, 124008 (2021)
[8] P. Morse, S. Roy, E. Agoritsas, E. Stanifer, E. Corwin, M. Manning, Proc. Natl. Acad. Sci. U.S.A., 118, (2021)
[9] E. Agoritsas, T. Maimbourg, F. Zamponi, J. Phys. A: Math. Theor., 52, 144002 (2019)
[10] G. Carleo, I. Cirac, K. Cranmer, L. Daudet, M. Schuld, N. Tishby, L. Vogt-Maranto, L. Zdeborová, Rev. Mod. Phys., 91, 045002 (2019)
[11] Elisabeth Agoritsas, Giovanni Catania, Aurélien Decelle, Beatriz Seoane, "Explaining the effects of non-convergent sampling in the training of Energy-Based Models", arXiv:2301.09428 [cs.LG] / ICML2023
[12] E. Agoritsas, G. Biroli, P. Urbani, F. Zamponi, J. Phys. A: Math. Theor., 51, 085002 (2018)
[13] Jascha Sohl-Dickstein, Eric A. Weiss, Niru Maheswaranathan, Surya Ganguli, "Deep Unsupervised Learning using Nonequilibrium Thermodynamics", arXiv:1503.03585 [cs.LG]
[14] Carlo Lucibello, Marc Mézard, "The Exponential Capacity of Dense Associative Memories", arXiv:2304.14964 [cond-mat.dis-nn]
[15] S. Goldt, M. Mézard, F. Krzakala, L. Zdeborová, Phys. Rev. X, 10, 041044 (2020)
[16] Miguel Ruiz-Garcia, Ge Zhang, Samuel S. Schoenholz, Andrea J. Liu, "Tilting the playing field: Dynamical loss functions for machine learning", ICML 2021 / arXiv:2102.03793 [cs.LG]
[17] A. Decelle, Physica A: Statistical Mechanics and its Applications, 128154 (2022)
[18] Chapter 24 to P. Charbonneau et al., "Spin Glass Theory and Far Beyond: Replica Symmetry Breaking after 40 Years", World Scientific (2023)
Practical information
- Informed public
- Registration required
Organizer
- Elisabeth Agoritsas (University of Geneva), Aurélien Decelle (Universidad Complutense de Madrid), Valentina Ros (CNRS and Université Paris- Saclay), Beatriz Seoane (Université Paris-Saclay)
Contact
- Aude Merola, CECAM Event and Communication Manager