Detecting Algorithmic Discrimination
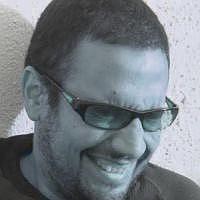
Event details
Date | 14.07.2017 |
Hour | 09:30 › 10:30 |
Speaker | Carlos Castillo http://chato.cl/research/ |
Location | |
Category | Conferences - Seminars |
Algorithms and decision making based on Big Data have become pervasive in all aspects of our daily (offline and online) lives, as they have become essential tools in personal finance, health care, hiring, housing, education, and policies. Data and algorithms determine the media we consume, the stories we read, the people we meet, the places we visit, but also whether we get a job, or whether our loan request is approved. It is therefore of societal and ethical importance to ask whether these algorithms can be discriminative on grounds, such as gender, ethnicity, marital or health status. It turns out that the answer is positive: for instance, recent studies have shown that Google’s online advertising system displayed ads for high-income jobs to men much more often than it did to women.
This algorithmic bias exists even when there is no discrimination intention in the developer of the algorithm. Sometimes it may be inherent to the data sources used (software making decisions based on data can reflect, or even amplify, the results of historical discrimination), but even when the sensitive attributes have been suppressed from the input, a well trained machine learning algorithm may still discriminate on the basis of such sensitive attributes because of correlations existing in the data.
From technical point of view, efforts at fighting algorithmic bias have led to developing two groups of solutions: (1) techniques for discrimination discovery from data and (2) discrimination prevention by means of fairness-aware data mining, develop data mining systems which are discrimination-conscious by-design. In this talk we mainly focus on the first groups of solutions: In the first part, we will present some examples of algorithmic bias, followed by introducing the sources of algorithmic discrimination, legal principles and definitions and finally measures of discrimination applied in fairness-aware data mining solutions. In the second part, we will introduce some of the recent data mining and machine learning approaches for discovering discrimination from the database of historical decision records.
This talk is joint work with Sara Hajian and Francesco Bonchi, and an extended version of it was presented as a KDD 2016 tutorial: http://francescobonchi.com/algorithmic_bias_tutorial.html
Carlos Castillo is Director of Research for Data Science at Eurecat. He is a web miner with a background on information retrieval, and has been influential in the areas of web content quality and credibility, and adversarial web search. He is a prolific researcher with more than 75 publications in top-tier international conferences and journals, receiving 9900+ citations. His works include a recent book on Big Crisis Data, as well as monographs on Information and Influence Propagation, and Adversarial Web Search.
Carlos received his Ph.D from the University of Chile (2004), and was a visiting scientist at Universitat Pompeu Fabra (2005) and Sapienza Universitá di Roma (2006) before working as a scientist and senior scientist at Yahoo! Research (2006-2012), and as a senior scientist and principal scientist at Qatar Computing Research Institute (2012-2015). He has served in the Program Committee (PC) or Senior PC (SPC) of all major conferences in his area (WWW, WSDM, SIGIR, KDD, CIKM, etc.), and is part of the editorial committee of Transactions on the Web and Internet Research. He is PC Co-Chair of ACM Digital Health 2017 and was PC Co-Chair of 2016, and of WSDM 2014; co-organized the Adversarial Information Retrieval Workshop and Web Spam Challenge in 2007 and 2008, the ECML/PKDD Discovery Challenge in 2010 and 2014, the Web Quality Workshop from 2011 to 2014, and the Social Web for Disaster Management Workshop in 2015 and 2016. He is an accredited advanced researcher (as required to be a full professor) by AQU in Catalonia, an ACM Senior Member, and an IEEE Senior Member.
Practical information
- General public
- Free
- This event is internal
Organizer
- Robert West https://people.epfl.ch/robert.west?lang=en
Contact
- Candice Norhadian https://people.epfl.ch/candice.norhadian?lang=en