Distributed Learning under Communication Constraints
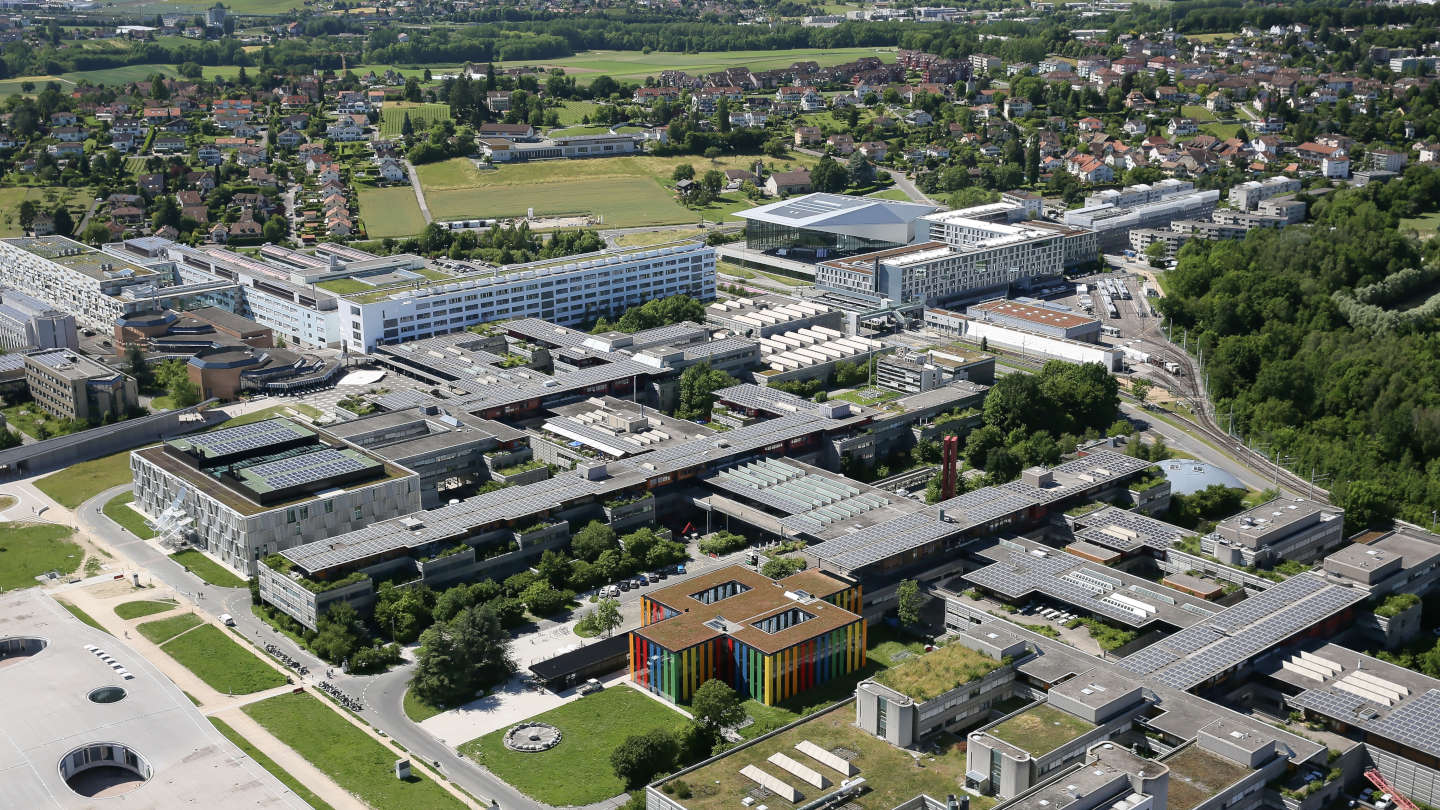
Event details
Date | 17.07.2019 |
Hour | 16:15 › 17:15 |
Speaker | Prof. Ayfer Özgur Aydin - Stanford University |
Location | |
Category | Conferences - Seminars |
We develop an information-theoretic framework for learning high-dimensional distributions and their parameters under communication constraints. We use this framework to prove tight minimax bounds for the distributed estimation of common statistical models (including the Gaussian location model, discrete distribution estimation, non-parametric estimation etc.) Our results reveal how the communication constraint impacts the estimation accuracy. We then use our theoretical framework to devise a communication-efficient distributed training algorithm for deep neural networks. Experimental evaluation suggests an order of magnitude improvement in communication efficiency over state of the art gradient compression algorithms.
Links
Practical information
- Informed public
- Free
Organizer
- IPG Seminar
Contact
- muriel.bardet@epfl.ch