NLP Seminar: An introduction to mechanistic interpretability
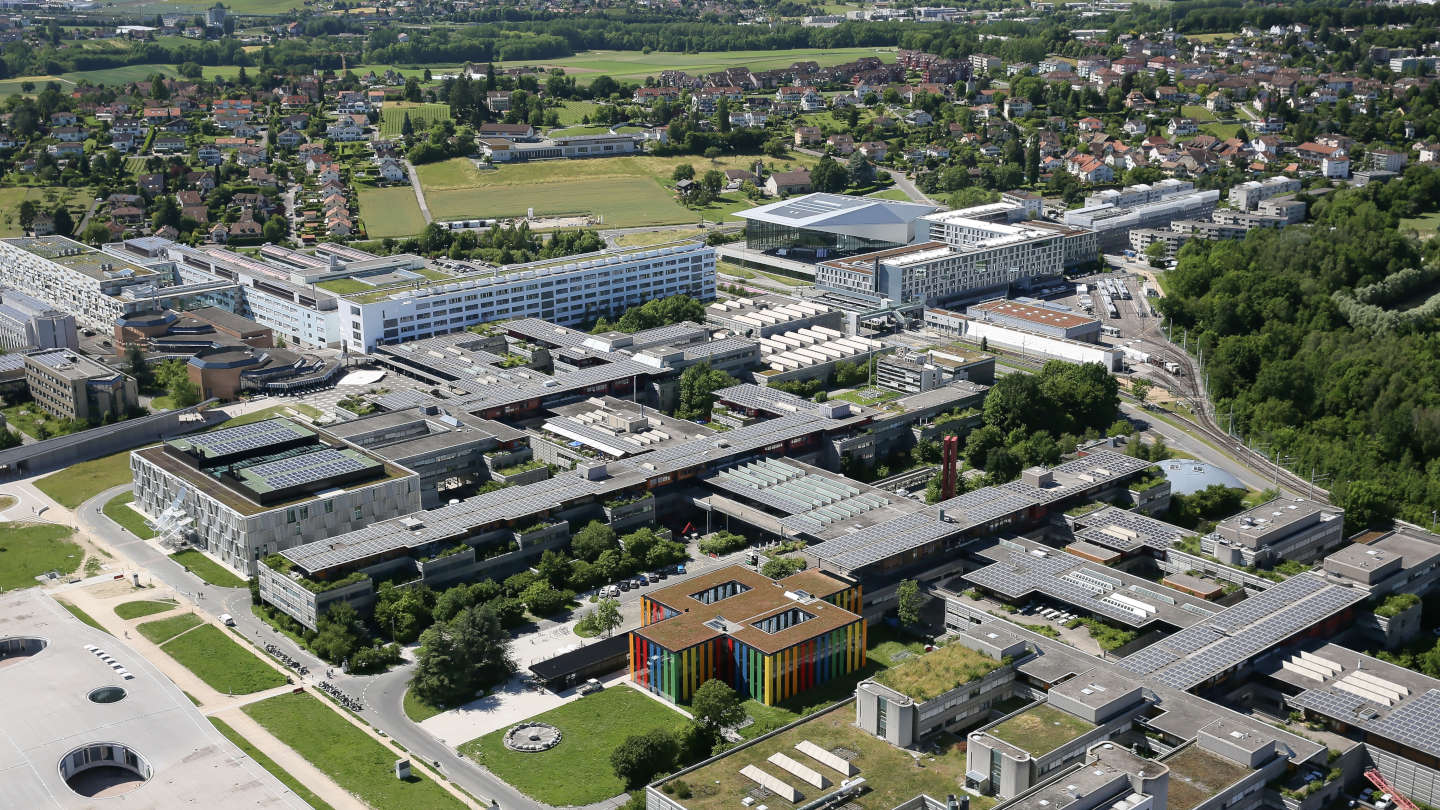
Event details
Date | 03.12.2024 |
Hour | 11:00 › 12:00 |
Speaker | Michael Hanna |
Location | Online |
Category | Conferences - Seminars |
Event Language | English |
Bio: Michael Hanna is a 3rd-year PhD student at the University of Amsterdam, as part of the Institute for Logic, Language and Computation. His research focuses on understanding the abilities of pre-trained language models, and linking these behaviors to low-level mechanisms using causal methods. He is particularly interested in understanding such language models' linguistic abilities, and their connection to human linguistic competence.
Abstract: Despite large language models' (LLMs) growing capabilities and increasing real-world deployment, our understanding of how they work remains limited: we don't know how they store facts, "reason", or even just produce grammatical language. Answering these questions could have wide-ranging impacts, from increasing model controllability to revealing insights into how human language works. In this lecture, I will provide an introduction to the field of mechanistic interpretability, which seeks to reverse-engineer LLMs by providing low-level, causally grounded explanations of the mechanisms that LLMs use to solve tasks. In particular, I will focus on two big questions often targeted by mechanistic interpretability, namely "Which parts of my model are responsible for a given task?" and "What features does my model use to solve this task?", as well as the solutions—circuit analysis and sparse autoencoders—that mechanistic interpretability has offered. By the end of this lecture, I aim to have provided you with all the tools necessary to begin your own investigation into what makes LLMs work!
Practical information
- Informed public
- Free
Organizer
- Antoine Bosselut, NLP lab