"Machine learning in chemistry and beyond" (ChE-651) seminar by Julian Zimmermann and Daniela Rupp "Machine learning based analysis of single-shot single-particle coherent diffraction imaging data"
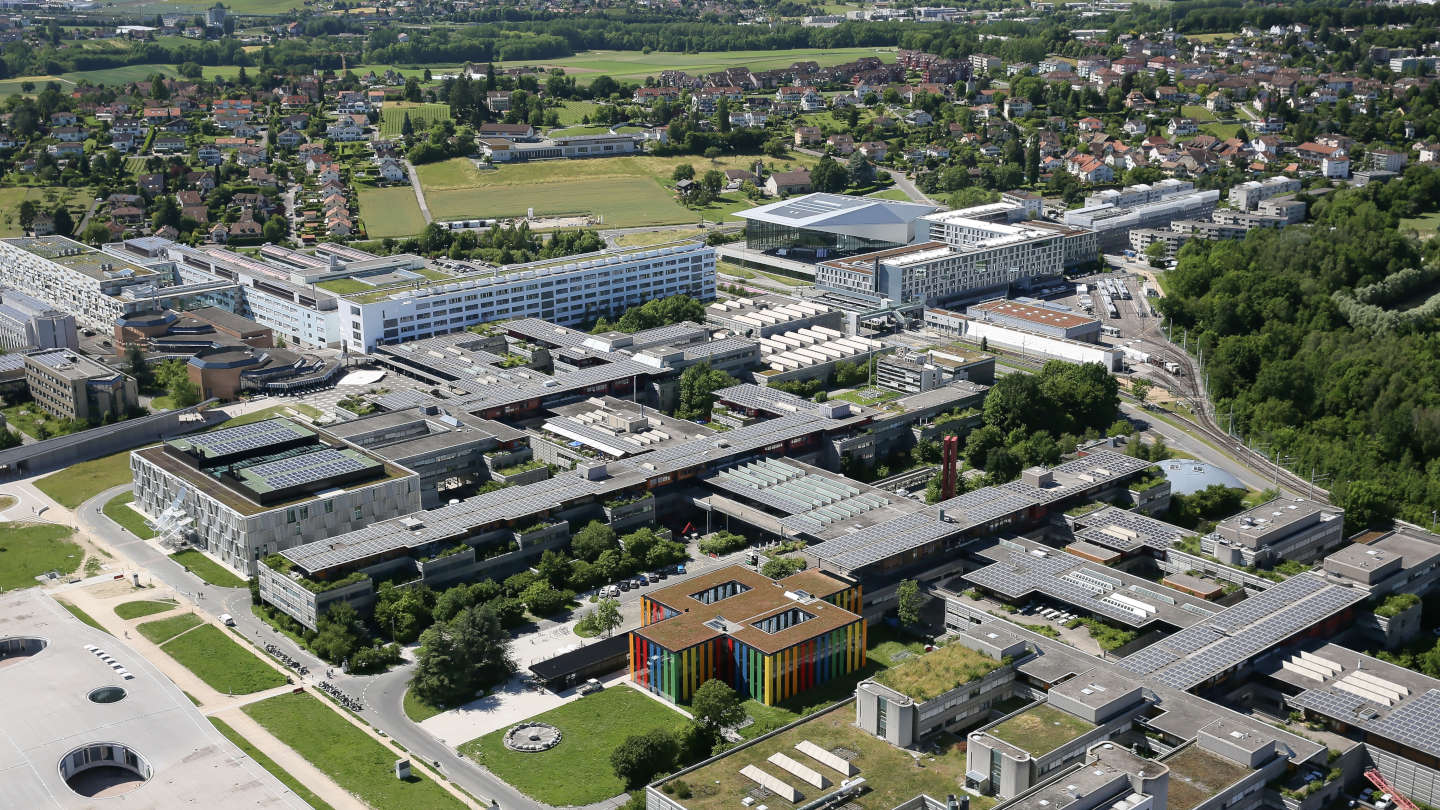
Event details
Date | 26.04.2022 |
Hour | 15:15 › 16:15 |
Speaker | Prof. Daniela Rupp is a Tenure-Track Assistant Professor at the head of the Nanostructures and Ultrafast X-ray Scienc group in the Department of Physics at ETHZ. Her group founded in 2019 develops and uses new methods to flash-image short-lived nanostructures and ultrafast changes in their electronic and structural properties via diffraction imaging, pushing towards extreme time-resolution. Before that, she was a Junior research group leader in "Ultrafast dynamics in nanoplasma" at the Max Born Institute in Berlin, Germany. She will present her research together with Dr. Julian Zimmermann, Postdoctoral researcher in her group at ETHZ. |
Location | Online |
Category | Conferences - Seminars |
Event Language | English |
With the extremely intense short-wavelength pulses of X-ray free-electron lasers (XFELs) and high harmonic sources (HHG) novel experiments with highest spatial and temporal resolution have become possible. One key example is coherent diffraction imaging (CDI) of individual nanoparticles.
Here, the elastically scattered photons form an interference pattern that encodes the structural information of a single particle in a snapshot. An example of such a diffraction pattern is given in the figure. The CDI method allows us to study such fragile structures as combustion aerosols or superfluid helium nanodroplets and “film” ultrafast dynamics like laser induced melting in metal nanoparticles. But in order to retrieve the structural and dynamical information, each pattern has to be decoded and a huge number of single diffraction patterns have to be analyzed. In that context, the tremendous progress of the last years in machine learning, especially for pattern and image recognition tasks, opens intriguing and long sought pathways for diffraction imaging analysis.
We adapt and apply supervised and self-supervised deep neural networks for image classification and similarity learning, significantly reducing the manual analysis tasks. In our talk, we will introduce the method of single-shot single-particle coherent diffraction imaging and explain the challenges we are facing and why they are calling for machine learning approaches. We will report on our developed, machine learning aided analysis pipeline and give an outlook on current and future machine learning applications in coherent diffraction imaging.
Practical information
- Informed public
- Free
Organizer
- Kevin Maik Jablonka, Solène Oberli, Puck van Gerwen
Contact
- Kevin Maik Jablonka, Solène Oberli, Puck van Gerwen