Prof. Fanny YANG: "Surprising failures of standard practices in ML when the sample size is small"
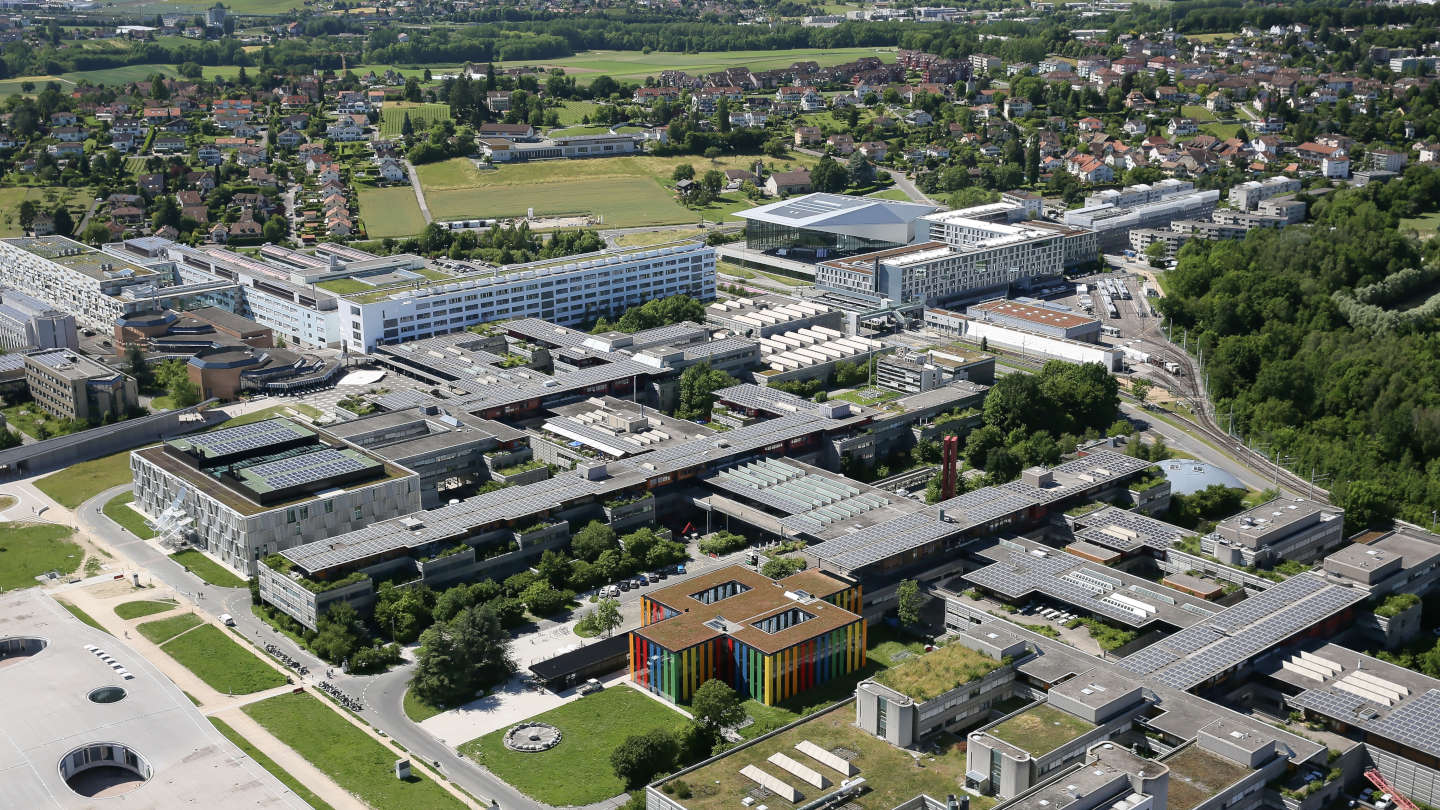
Event details
Date | 30.10.2023 |
Hour | 10:30 › 11:30 |
Speaker | Prof. Fanny Yang - ETHZ |
Location | |
Category | Conferences - Seminars |
Event Language | English |
Abstract:
In this talk, we discuss two failure cases of common practices that are typically believed to improve on vanilla methods: (i) adversarial training can lead to worse robust accuracy than standard training (ii) active learning can lead to a worse classifier than a model trained using uniform samples. In particular, we can prove both mathematically and empirically, that such failures can happen in the small-sample regime. We discuss high-level explanations derived from the theory, that shed light on the causes of these phenomena in practice.
Bio:
Fanny Yang is an Assistant Professor of Computer Science at ETH Zurich. She received her Ph.D. in EECS from the University of California, Berkeley in 2018 and was a postdoctoral fellow at Stanford University and ETH-ITS in 2019. Her current research interests include methodological and theoretical advances for problems that arise from distribution shifts and hidden confounding, and studying the (robust) generalization ability of overparameterized models for high-dimensional data.
In this talk, we discuss two failure cases of common practices that are typically believed to improve on vanilla methods: (i) adversarial training can lead to worse robust accuracy than standard training (ii) active learning can lead to a worse classifier than a model trained using uniform samples. In particular, we can prove both mathematically and empirically, that such failures can happen in the small-sample regime. We discuss high-level explanations derived from the theory, that shed light on the causes of these phenomena in practice.
Bio:
Fanny Yang is an Assistant Professor of Computer Science at ETH Zurich. She received her Ph.D. in EECS from the University of California, Berkeley in 2018 and was a postdoctoral fellow at Stanford University and ETH-ITS in 2019. Her current research interests include methodological and theoretical advances for problems that arise from distribution shifts and hidden confounding, and studying the (robust) generalization ability of overparameterized models for high-dimensional data.
Practical information
- Informed public
- Free